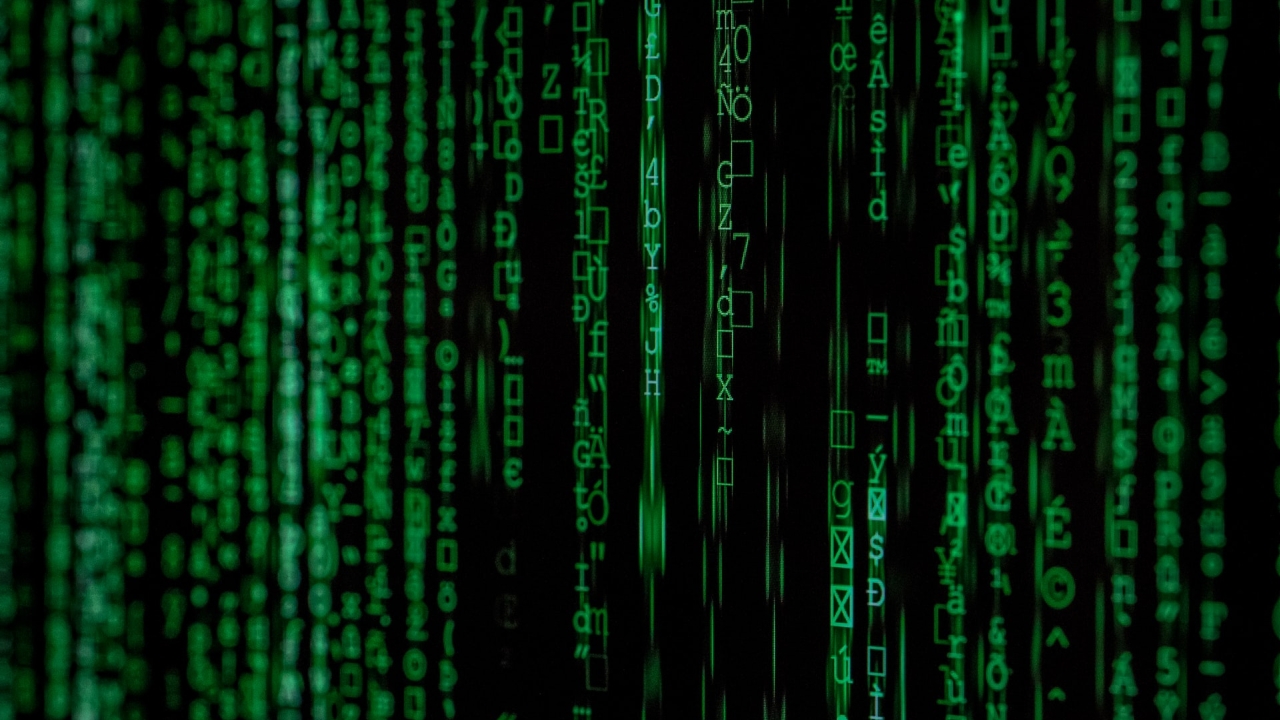
How knowledge management systems can be reformed to bring big data into strategic decisions
Big data1 primarily refers to data sets that are too large or complex to be dealt with by traditional data-processing application software. However, despite these analysis difficulties, big data has been attracting increasing interest from knowledge management (KM) practitioners, and has been identified as one of eight emerging innovative concepts in KM2.
Supporting the importance of big data in KM,
- organization data is one of the four sources of evidence in evidence-based practice
- the international knowledge management (KM) standard ISO 30401:2018 Knowledge management systems – Requirements identifies technology as a KM enabler, and one of the important uses for this technology is data processing
- the analysis of big data can bring considerable additional benefits beyond what can be learnt from basic datasets.
A recent paper3 published in the Journal of Knowledge Management alerts that to extract information from big data that is of value to an organization, new techniques and advanced tools have to be developed and applied, such as advanced data mining4 or new artificial intelligence5 tools.
This can be challenging, especially when an organization wants to get the most out of its current expensive KM systems by expecting its KM systems to handle big data as well. To utilize these resources and fulfill the consequently advanced requirements, paper authors Ali Intezari and Simone Gressel advise that the existing systems need to be reformed and upgraded to “advanced KM systems.”
To assist organizations to do this, Intezari and Gressel suggest a conceptual framework for how KM systems can incorporate big data into strategic decisions. To develop the framework, they analyzed the KM and data analysis systems implemented by organizations, complemented by a review of relevant research literature.
As a foundation for their research and conceptual model, Intezari and Gressel reinforce the point that data is not knowledge. From an organizational perspective, while data is found in records, knowledge derives from minds at work and develops over time through experience. As with knowledge, return on big data is associated with making decisions cheaper, faster, and better than before. However, while big data and advanced analytics have the potential to add value by providing transparency through immediate performance feedback and more objective decision-making, effective formulation and implementation of strategic decisions is not just the result of having access to big data and cheap databases.
The characteristics of big data
Intezari and Gressel advise that big data is now commonly specified by three V’s, which serve as a distinction between big data and traditional data sets – volume, velocity, and variety – with recent literature also suggesting the addition of a fourth, veracity:
- The volume of big data exceeds the size of regular data sets by far, and creates challenges
for traditional database management systems and data warehouses in terms of data storage and analysis. This increase in data volume is attributed to the continuous growth of data that is produced every second over the internet, sensors, customer transactions, and so forth. - The velocity of data is characterized by the speed of data creation and analysis. Regarding velocity, a data set can only be classified as big data if the data are processed in real-time or near real-time.
- The variety of big data refers to the different sources and types of data that are stored. Data is not limited to structured, numerical data anymore. It is gathered in unstructured forms from social networks, texts, audio or video files, sensor data, GPS signals, and so on.
- The veracity factor assesses how credible a data source is and how well the data suits the organization’s audience.
As defined by the variety characteristic above, big data refers to both structured and unstructured data. Big data technologies enable companies to gain insights from diverse data sources that outperform the traditional internal and structured data that organizations relied on in the past. Traditionally, organizations relied on data that was stored in their relational databases and easily queried. In contrast, the rise of unstructured data sources poses new challenges for the integration of different data types.
Unstructured data, such as social media data, can provide an in-depth insight into human behavior, as can be seen in the example of Twitter data. However, Intezari and Gressel caution that the reliability and quality of social media data can vary greatly. In the example of Twitter, not all contributions are user-generated, with some posted by automated programs, therefore compromising insights.
A notable example of over-reliance on big data, and specifically unstructured data, for predictive analytics is Google Flu Trends. On several occasions, Google Flu Trends managed to surpass predictions from the Centres for Disease Control and Prevention. However, in the long run, it produced large errors in its predictions by overlooking information that would have been attainable by using traditional statistical methods.
The characteristics of decision-making
As with big data, Intezari and Gressel discuss how there can be both structured and unstructured decision-making. This depends on the level of the complexity of the problem, for example as highlighted by the Cynefin framework6.
Structured decisions are made through orderly or sequential processes, and can be described by classic mathematical models (e.g. statistical methods and linear programing). On the other hand, there is no standard and global method for obtaining an optimal solution to address unstructured decision problems.
Unstructured decisions refer to the decision processes that have not been encountered in quite the same form and for which no predetermined and explicit set of ordered responses exists in the organization. Unstructured decision problems are vague, uncertain and fuzzy, for which no pre-defined process and optimal solution exists. This means that human intuition, experience and judgement are often the basis for the decision-making.
Intezari and Gressel note that advocates of unstructured decision-making would strongly argue that managers do not necessarily make decisions by following clearly structured and pre-defined phases. Instead, they make their decisions on the basis of a combination of data, experience and feeling. Decision-making is not necessarily always a “thinking first” process. There is also no clear-cut border between the two processes, and it is unlikely that an organization would use only one type of decision-making.
Conceptual model
Using the conceptualizations of structured and unstructured data and structured and unstructured decision-making (DM) described above, Intezari and Gressel have prepared the conceptual model shown in Figure 1 and elaborated after the figure.

Structured decisions based on structured data (SD-SD)
An organization may have structured processes and pre-defined procedures to make strategic decisions based on structured data. Following instructions and pre-defined processes is critical to make effective decisions. Structured decisions made based on structured data (SD-SD) can be formulated using mathematical modeling, which represents mathematical relationships between variables (e.g. explanatory models for forecasting or algebraic models for optimization). Furthermore, advanced analytics can be applied to make automated decisions based on gathered and organized data.
Structured decisions based on unstructured data (SD-UD)
“Weak signals” from social media and other sources contain powerful insights and should be part of the data-creation and decision-making process. The decisions that are made based on unstructured data but through pre-defined procedures are in this category. Although applying models to utilize inherent patterns and structures in unstructured data might be challenging, techniques such as mathematical modeling can be used in SD-UD. Compared to SD-SD, SD-UD may require additional steps to turn unstructured data into structured data.
Unstructured decisions based on structured data (UD-SD)
Decision-making as an unstructured process suggests that the steps of decision-making do not necessarily follow a set order of tasks. Unstructured decisions rely more on human judgement, experience, prior knowledge, and interpretation of the decision context and alternatives. UD-SD is when the individual decision maker or the organization has access to structured data, but there are little or no clear and pre-defined decision-making procedures to follow to integrate the data into the decision.
Unstructured decisions based on unstructured data (UD-UD)
Incorporating unstructured data, such as social media data, into strategic decisions can be very challenging when there is little or no clear decision procedure or structure. Compared to the other decision-data quadrants, UD-UD is the least structured data-based decision. As unstructured decisions are often made to address unstructured and complex problems, context analysis is critically important. Social interactions play a critical role in UD-SD and UD-UD types of decision-making, and although data-driven decision support techniques can be useful in all four decision-data quadrants, UD-UD relies mainly on human interpretation and insight, rather than mathematical analysis.
Ground rules for advanced KM systems
To support all four of the data-driven decision types above, Intezari and Gressel state that KM systems need to accommodate both data analytics and human insight. They suggest five key features that characterize these advanced KM systems: social, cross-lingual, integrative, dynamic and agile, as well as simple and understandable.
They alert that little attention has been paid to the interconnectedness of all of these features, and so argue that they should not be considered in isolation but rather incorporated into KM systems as integrated features aligned with organizational strategic decisions.
Social
Although the roles of big data and advanced analytics in enhancing business decisions are undeniable, only human managers (not machines) can decide on critical issues. Knowledge is built on know-how and develops based on the person’s own experiences, perceptions, preferences, perspectives, values, and beliefs. Accordingly, sharing knowledge requires other mechanisms such as social networks rather than standard databases and procedures.
Social media and Web 2.0 technologies fundamentally change the way employees handle knowledge processes such as knowledge creation, sharing, and implementation. Many social media platforms attract users with diverse interests and offer easy search facilities that enable the users to find the most relevant expertise. “Microblogging” is an example of the features that advanced KM systems can offer to facilitate knowledge sharing through social interactions.
That connecting “people to people” must be part of all KM systems is not a new thing. However, in advanced KM systems, the level of social interaction is determined by the type of the data-driven decisions. While data analytics underlie SD-SD and SD-UD, social interactions play a critical role in UD-SD and UD-UD by evaluating and integrating big data and human insight.
Advanced KM systems not only encourage and facilitate social interactions within the organization but also support interactions between the organization and outside stakeholders.
Cross-lingual: cross-lingual knowledge retrieval
Given the globalization and increasing multi-cultural and multi-national work environments in today’s businesses, the “social” feature of KM systems is augmented by another feature that supports interaction among people with different languages: “cross-lingual.”
Over 150 languages are used on the internet. This indicates that information systems, and more particularly, KM systems, need to be able to handle cross-lingual interactions by offering an effective cross-lingual knowledge retrieval (CLKR) feature.
Cross-lingual information retrieval (CLIR) – also referred to as multi-lingual information retrieval, which is mainly concerned with data and information, will be complementary to CLKR. The CLKR-CLIR combined feature allows for advanced KM systems to be able to explore, store and retrieve data, information, and knowledge.
Drawing on an integrated feature of CLKR-CLIR, advanced KM systems can support all the decision types of SD-SD, UD-SD, SD-UD and UD-UD. CLKR enhances the integration of advanced KM systems.
Integrative
CLKR allows an advanced KM system to enable its users to freely and in a controllable manner open up their knowledge, expertise, insight, experiences, expectations, perceptions, personal and professional perspectives, values, and beliefs either generally or regarding a specific matter.
The users can assess the knowledge not only in regard to the subject matter or the decision context but also in association with the participant’s personality and professional background and expertise. This way, users are more capable of and have more control over sharing and absorbing knowledge across a network of users within and outside the organization. The multi-dimensional assessment of knowledge leads to what is called “knowledge fit.”
A KM system characterized as “integrative” assists with capturing and aggregating the data and knowledge that are fragmented and scattered across the organization, and drawing meaningful connections between structured and unstructured data.
Furthermore, the output of the KM systems should not be limited to textual outputs: it should also support non-textual expertise, for example, visual reasoning.
Dynamic and agile
The social, cross-lingual and integrative aspects lead advanced KM systems to be dynamic and agile. To make relevant and viable decisions, dynamism and agility are vital in the decision-making process.
The primary objective of exploring big data is to make decisions in real-time. Advanced KM systems must be able to handle the velocity of the available data by allowing for collecting, aggregating, and sharing the data and the knowledge generated during the interaction of the participants. This is particularly important as the speed of strategic decision-making is directly related to performance. The fast pace of data generation provides an opportunity to incorporate real-time data and information into decision-making.
However, faster analysis of data does not necessarily lead to an effective decision if the required expertise, experience, knowledge, and insight are not made available to interpret and integrate the data analysis into the decision. While SD-SD and SD-UD may exhibit more agility, UD-SD and UD-UD are more dynamic. Accordingly, KM systems need to be both dynamic and agile.
Simple and understandable
For the KM systems to be able to benefit from agility and dynamism, the systems must be simple and understandable for people throughout the organization. Advanced KM systems must be designed in such a way that the decision maker and all other participants involved in the KM system are able to understand the tool and, more importantly, have confidence in it.
While developing algorithms to support SD-SD may require a high level of analytical and technical skills, UD-UD and UD-SD rely more on expert knowledge and insight. The need for the engagement of a diverse range of users with different knowledge and skills in advanced KM systems toward supporting all four types of decisions may lead the KM system to be complicated. Unnecessary complication of the system reduces the likelihood and level of user participation.
However, designing and developing advanced KM systems is not simple and may be significantly challenging. Bringing together a diverse range of users such as data analysts, managers, business experts and strategy analysts may lead to a KM system that may not offer all the features of an advanced KM system to the extent that has been discussed above, instead offering moderate and satisfactory features.
Intezari and Gressel emphasize that the design of the KM systems may vary depending on factors such as organizational objectives and strategies, users’ preferences and organizational culture. They suggest that while the ground rules above are regarded as necessary components of advanced KM systems, the extent to which an organization places emphasis on any of the features should reflect the organizational objectives and culture, as well as users’ preferences.
See also: Combining human and artificial intelligence for organizational decision-making under uncertainty.
Header image source: Markus Spiske on Unsplash.
References:
- Wikipedia, CC BY-SA 3.0. ↩
- Schenk, J. (2023). Innovative Concepts within Knowledge Management. Proceedings of the 56th Hawaii International Conference on System Sciences, 4901-4910. ↩
- Intezari, A., & Gressel, S. (2017). Information and reformation in KM systems: big data and strategic decision-making. Journal of Knowledge Management, 21(1), 71-91. ↩
- Peu, E. (2021). Automated Knowledge Discovery or Integration: A Systematic Review of Data Mining in Knowledge Management. (Master’s dissertation, Stellenbosch University). ↩
- Jarrahi, M. H., Askay, D., Eshraghi, A., & Smith, P. (2023). Artificial intelligence and knowledge management: A partnership between human and AI. Business Horizons, 66(1), 87-99. ↩
- Snowden, D. J., & Boone, M. E. (2007). A leader’s framework for decision making. Harvard Business Review, 85(11), 68. ↩
Also published on Medium.