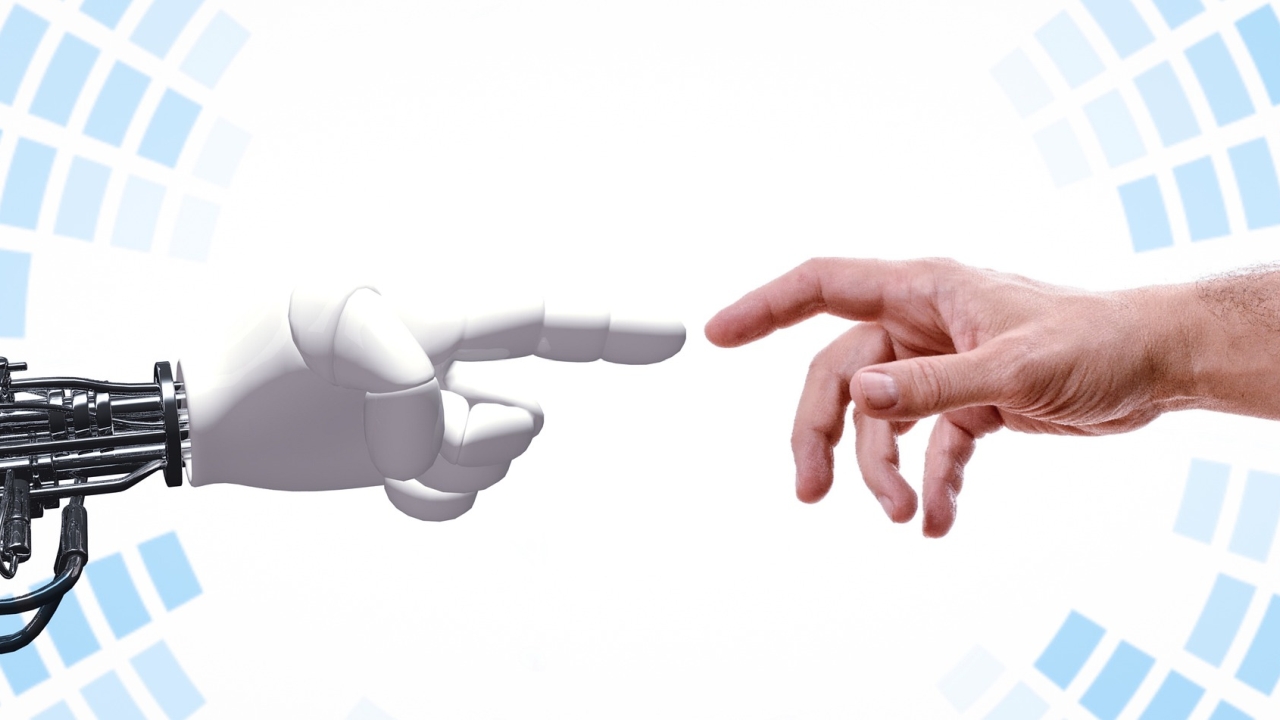
How artificial intelligence can support knowledge management in organizations
The potential for artificial intelligence (AI) to play a role in supporting knowledge management (KM) activities is attracting considerable interest, with AI identified as one of eight emerging innovative concepts in KM1.
A newly published paper2 in the Business Horizons academic journal aims to uncover opportunities for the use of AI in KM. In doing so, paper authors Mohammad Hossein Jarrahi, David Askay, Ali Eshraghi, and Preston Smith explore three areas:
- The potential role for AI in supporting fundamental dimensions of KM: creation, storage and retrieval, sharing, and application of knowledge.
- Practical ways to build the partnership between humans and AI in supporting organizational KM activities.
- Implications for the development and management of AI systems based on the components of people, infrastructures, and processes.
1. Potential applications of AI in KM
Jarrahi and colleagues first discuss some potential implications of AI systems for KM, including examples, as shown in Table 1. The KM processes in Table 1 are those identified by Maryam Alavi and colleagues3, being creating, storing and retrieving, sharing, and applying knowledge.
The KM process | Possibilities created using AI systems | Examples of use cases |
Knowledge creation |
|
|
Knowledge storing and retrieving |
|
|
Knowledge sharing |
|
|
Knowledge application |
|
|
2. Human–AI symbiosis in KM
Although AI systems will likely percolate throughout the KM foundation of organizations, Jarrahi and colleagues remind us that that knowledge production and management are inherently human-centered.
Therefore, they propose that the most effective roles assigned to AI in KM will mostly augment humans rather than replace them, thereby achieving collaborative intelligence in which AI and humans enhance each other’s complementary strengths. This also aligns with the findings of a previous paper4 that explored combining humans and AI for organizational decision-making under uncertainty.
Some potential scenarios and practical ways for building the human-AI partnership are shown in Figure 1, and elaborated after the figure.
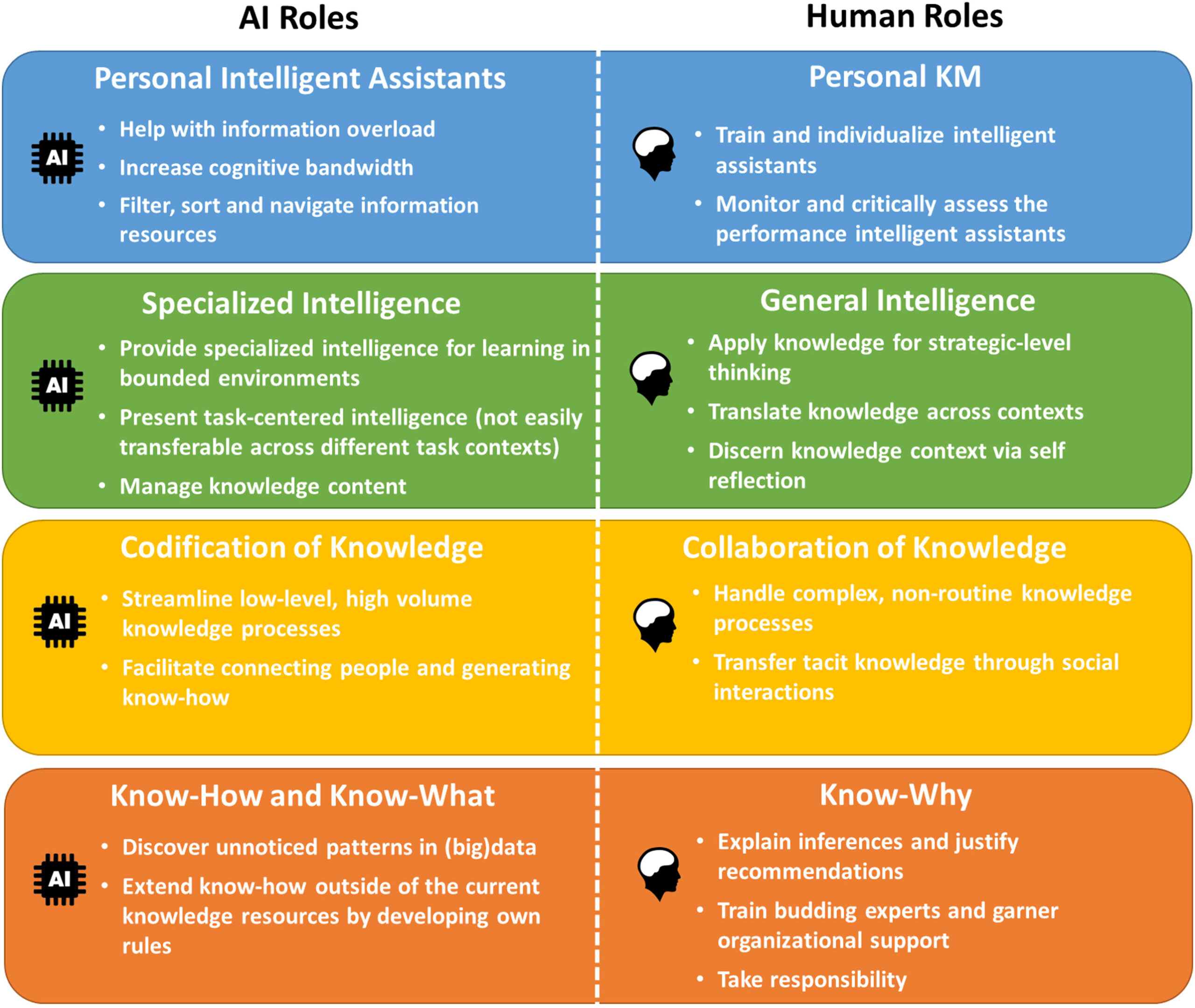
Personal intelligent assistants and personal KM
Conceptualizations of knowledge often recognize the centrality of each worker in defining the concept of knowledge. An emerging genre of AI systems called personal intelligent assistants can occupy a unique position in personal KM.
Information overload is one of the key challenges of the information environment for knowledge workers. Personal intelligent assistants can help broaden the cognitive bandwidth of knowledge workers and change the way they digest relevant knowledge by providing more effective capabilities for processing, filtering, sorting, and navigating information resources.
Humans are still central in shaping the effectiveness of personal intelligent assistants, as these systems thrive on the worker’s feedback and interaction with the information they provide.
Specialized intelligence versus general intelligence
AI presents specialized intelligence that enables sensing the environment, learning from experience, and creating possibilities for action in relation to specific task contexts. General intelligence, however, remains a human-centered characteristic.
Specifically, the application of knowledge for strategic-level thinking and decision-making requires elements of general intelligence, and builds from the uniquely human prerogatives such as foresight, social and emotional intelligence, self-development, imagination, and curiosity.
Furthermore, KM entails a crucial judgment component, which is a profoundly human-centered capability. Finally, a central attribute of general intelligence is self-reflection, which perpetuates the need for self-awareness about one’s knowledge regarding areas of strength and weakness.
Codification versus collaboration
Two dimensions of KM can be supported by IT uses: codification of knowledge and human collaboration. In regard to codification, AI systems can streamline the tasks of collecting, classifying, analyzing, and presenting content, and in doing so, free up knowledge workers for higher value-added tasks.
In regard to collaboration, AI technologies provide great capabilities for generating know-who (i.e., sources of expertise) within and across organizational boundaries and for extending and augmenting knowledge networks. But transferring tacit knowledge remains a highly human-centered practice, and attempts to turn inherently tacit knowledge into explicit knowledge and to facilitate its transfer through technological mechanisms have failed in the past.
An example of an AI system that harnesses the unique contributions of both humans and machines is Swarm AI, which enables groups of humans to collectively converge on a decision in real time.
Know-how and know-what versus know-why
In traditional KM systems, algorithms were precoded with rules created by humans, and through defined processes, could transform standard inputs into standardized outputs.
In contrast, AI systems can now self-learn to develop and improve know-how and know-what, offering more effective outputs as they process new data. However, this self-learning makes it difficult, if not impossible, to explain the inferences generated by the black box of AI. This is a particularly serious problem in evidence-based fields such as medicine and law, where there are clear obligations for explaining how AI systems may weigh the inputs they receive to inform certain recommendations.
Therefore, the role of humans is indispensable in formulating know-why for AI-based inferences; know-why is essential for alleviating the black box of AI, justifying decisions, training budding human experts, and garnering organizational support.
3. Practical implications
A recent survey indicates that the vast majority of AI investments have little or no impact. But years of research indicates that for an IT deployment to be successful, there need to be accompanying organizational changes. These are referred to as organizational complements.
So Jarrahi and colleagues advise that the value of AI for KM lies not only in technology, but also in new infrastructures, trained people, and redesigned processes. These are shown in Figure 2, and elaborated after the figure.
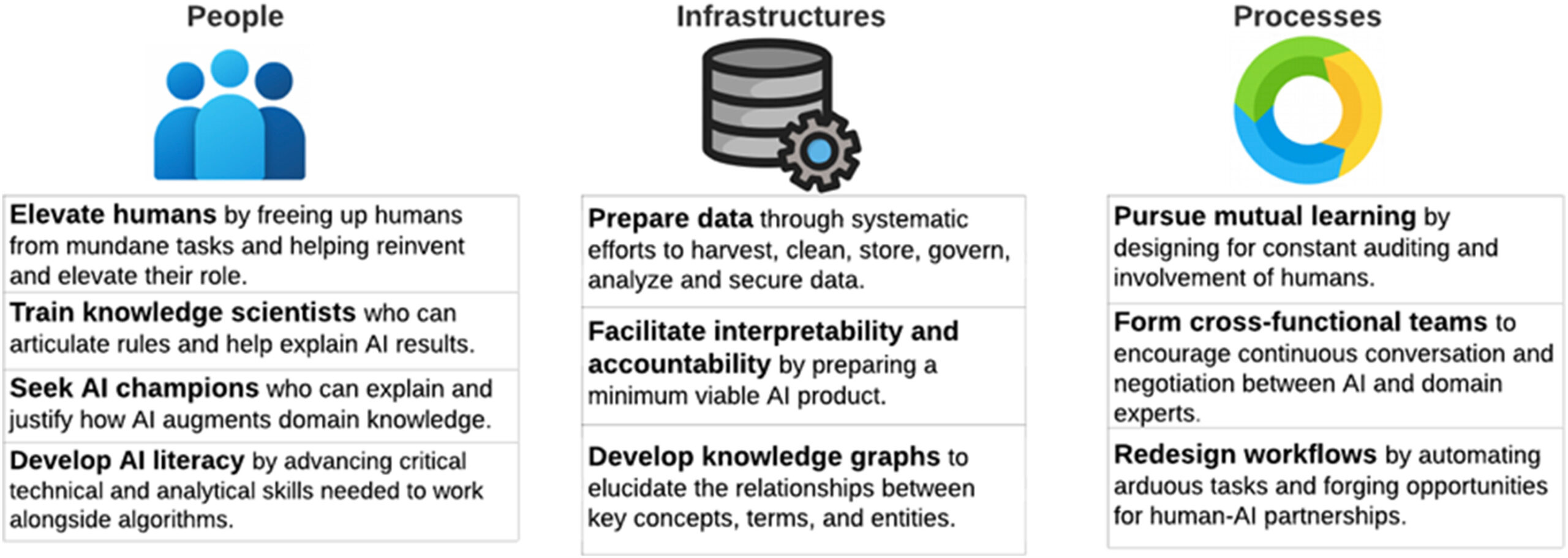
People
- Elevate humans in KM – Humans are often an afterthought in AI implementation, seen as an appendage to the technological AI system or as a roadblock to full automation. Instead, Jarrahi and colleagues argue for a symbiotic relationship, one that both recognizes the irreplaceable contributions of humans to knowledge work and that seeks ways to reinvent and elevate their role. One way in which AI can elevate knowledge workers is through reskilling and upskilling.
- Train knowledge scientists – Recent developments in explainable AI (XAI) or neural-symbolic learning indicate the need for new roles, such as knowledge scientists and data scientists, who collect and prepare training data sets for machine learning algorithms. Knowledge scientists can contribute to the process of combining the two distinct AI strategies – symbolic AI (using more traditional approaches) and statistical AI (based on neural networks) – by helping to build knowledge graphs that represent background knowledge and that complement training data. Given their understanding of the KM context and their close interaction with the AI system, knowledge scientists are also central in articulating know-why, at least in some degree.
- Seek AI champions – AI champions can be instrumental in presenting an alternative narrative that emphasizes augmenting knowledge workers rather than replacing them – an alternative narrative that describes the expected improvement in the kinds of tasks knowledge workers perform. AI champions are boundary spanners who find correspondence between specific AI capabilities and specific KM needs and functions. While in some cases, new people need to be hired with the right cognitive skills and analytical backgrounds, current employees generally must be trained and retrained to occupy these new positions.
- Foster AI literacy – As AI automates repetitive and mundane tasks that were performed by humans, workers will need to learn how to interact with intelligent systems rather than with humans for many of these tasks. AI literacy is a key component needed for upskilling both managers and workers interacting with AI systems. This requires knowledge workers to develop a fuller appreciation of their artificial counterparts, algorithmic competencies, and new analytical, data-centered skills that help workers interpret AI-based decisions.
Infrastructure
- Prepare data – The new generation of AI is powerful partly owing to the abundance of data generated in contemporary organizations. Deep-learning approaches, for example, require rather large sets of training data to produce reliable outcomes. Hence, quality and quantity of data are key success factors of deep-learning systems.
- Facilitate interpretability and accountability – AI technology itself also needs to be adapted for organizational rollout. Many of the AI capabilities developed in academic and corporate labs may not be ready to be deployed in knowledge practices owing to the issues of interpretability and accountability.
- Develop knowledge graphs – One of the key challenges of leveraging knowledge has to do with the fact that data are dynamically being generated in real time, and most such data are unstructured. This presents barriers to timely and meaningful AI-empowered analysis. Knowledge graphs are an emerging way in which organizations can harness this data. They manage the key concepts, terms, and entities and their relationships in the business. Many businesses already use knowledge graphs for AI-enabled KM.
Processes
- Pursue mutual learning – Process redesign must both draw on and facilitate mutual learning between humans and AI. Designing for mutual learning recognizes the limits of the AI system in managing knowledge and precipitates the need for constant auditing and involvement of human supervisors. Datafication and digitalization, coupled with the use of AI, can give the wrong impression of authentic knowledge, but overestimating or trusting too much in the learning capacities of AI may thwart the real value of these systems for KM.
- Form cross-functional teams – AI capabilities can themselves be used to re-engineer existing organizational processes. Algorithmic systems thrive on comprehensive data that span multiple units, so providing this data for AI systems requires organization-wide initiatives, which could be facilitated by interdisciplinary and cross-functional teams. The redesign of workflows and the identification of ways in which algorithms’ recommendations can augment various knowledge activities require a continual conversation and negotiation between technology and domain experts. Such a process cannot be performed top-down.
- Redesign for automation and augmentation – Automation is not necessarily the opposite of human augmentation and can in fact foster augmentation. Elevating humans necessitates that organizations look for opportunities to free knowledge workers from arduous and monotonous work by automating it. Some of this repetitive work can be delegated to intelligent assistants. These intelligent systems can extend their reach into exception handling. When redesigning processes, organizations also need to simultaneously consider opportunities for augmentation.
Article source: Artificial intelligence and knowledge management: A partnership between human and AI, CC BY 4.0.
Header image source: Mohamed Hassan on Pixabay, Public Domain.
References:
- Schenk, J. (2023). Innovative Concepts within Knowledge Management. Proceedings of the 56th Hawaii International Conference on System Sciences, 4901-4910. ↩
- Jarrahi, M. H., Askay, D., Eshraghi, A., & Smith, P. (2023). Artificial intelligence and knowledge management: A partnership between human and AI. Business Horizons, 66(1), 87-99. ↩
- Hashim, S., Mohamad, M. M., & Muda, W. H. N. W. (2018). Knowledge construction models: A view of various models for an impeccable learning environment. In MATEC Web of Conferences (Vol. 150, p. 05013). EDP Sciences. ↩
- Trunk, A., Birkel, H., & Hartmann, E. (2020). On the current state of combining human and artificial intelligence for strategic organizational decision making. Business Research, 13(3), 875-919. ↩
Also published on Medium.