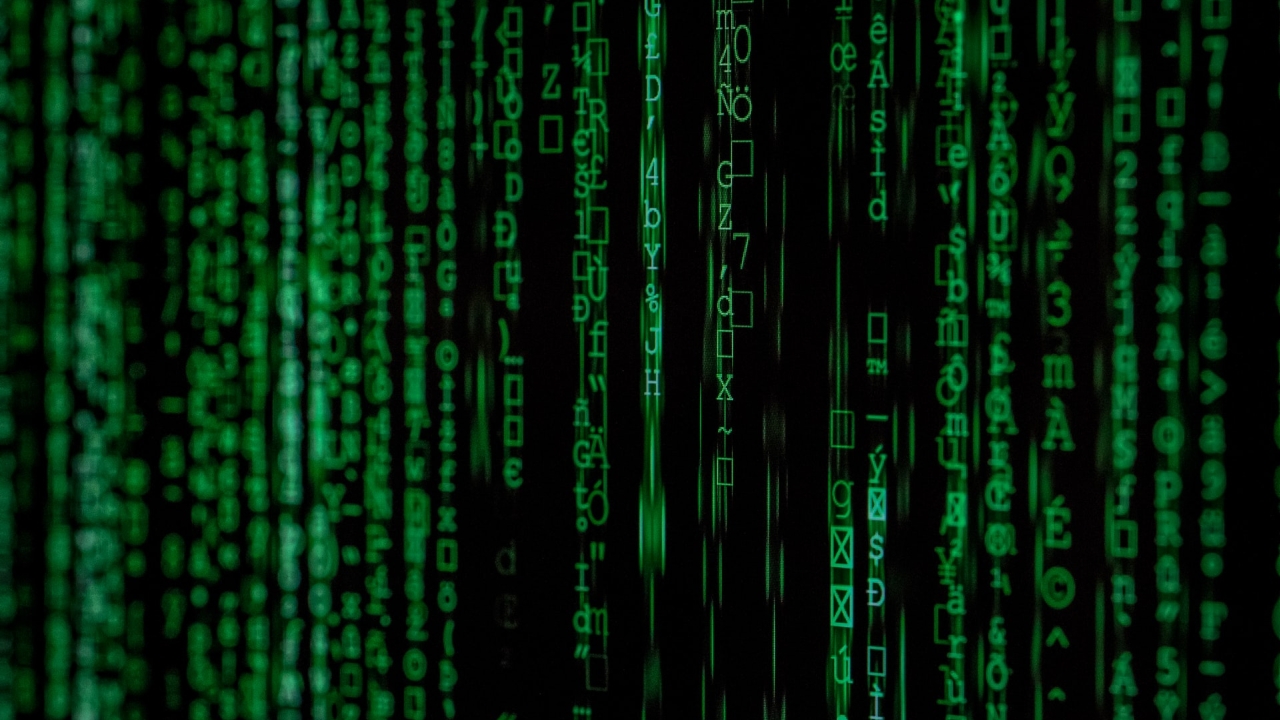
What is the role of data mining in knowledge management?
The international knowledge management (KM) standard ISO 30401:2018 Knowledge management systems – Requirements identifies technology as one of a number of KM enablers (while also reminding us that a technology system alone will not be enough for KM to add value).
One of the ways in which technology can be applied as a KM enabler is to use it to facilitate data mining, with organisation data being one of the four sources of evidence in evidence-based practice.
Ephenia Peu explores the role of data mining in KM is a recently completed Stellenbosch University Master of Philosophy (Information and Knowledge Management) dissertation1. To do this, Peu analyses the results of a systematic literature review which was carried out using the PRISMA model2.
The systematic literature review identified 54 relevant articles eligible for inclusion in the analysis. Six themes were then identified in a thematic analysis. The findings from the reviewed literature for each of the themes provides useful introductory guidance in regard to data mining in KM:
1. Advances in ICT facilitate access to and transformation of knowledge for better performance
Information and communication technology (ICT) enables better access to knowledge in an organisation. ICT also supports the integration of other systems in an organisation and enhances the collaboration and interaction between those systems.
ICT systems facilitate organising, formalising, and distributing information that may be converted to knowledge using current components of an organisation’s ICT infrastructure such as databases and communication technologies. For example, the internet, corporate extranets, and company portals primarily support the objective of making it easy to find and re-use sources of know how and expertise. Sensors and embedded technology also now enable the transmission of real-time data from wireless networks, which can lead to the co-creation of new real-time knowledge among customers and vendors.
2. A knowledge base as a tool for improved competitive advantage and product and service development
The availability and accessibility of a platform such as a knowledge base improves the expertise of staff to solve problems and supports the enhanced development of products and services, providing a competitive edge for the organisation. A knowledge base system supports product customisation and rapid innovation in development and product configuration. It allows an effective and efficient processing of knowledge transactions during product realisation.
3. The emergence of big data analytics for customer relationship management (CRM)
Appropriate customer relationship management (CRM) means:
- presenting an image of unity of the organisation
- knowing who your customers are and what their preferences and dislikes are
- preempting the needs of the customer and proactively addressing them
- acknowledging customers dissatisfaction and taking corrective action.
Emerging technologies can generate data about customer behaviour, sales, and buying patterns, including data that can be used to develop marketing strategies. An aspect of this is segmentation, which enables the organisation to provide more personalised and therefore more attractive product and service offerings to individual customer groups. Criteria for segmenting customers include customer profitability score, retention score, satisfaction and loyalty score, and response to promotion.
Big data technology can be used to analyse social media data related to the organisation and its competitors, and to visualise and benchmark comparisons among competitors across events, products, issues, and any other areas that may affect business performance.
4. Understanding data, information and knowledge assets as interlinked capabilities for decision support
It is important to know what intangible assets are available in an organisation, especially what knowledge assets are available and accessible. Metrics can be used to understand the contribution of different intangibles (big data/information, knowledge, intelligence) to competitiveness in particular organisations and in specific industries. This can assist in determining what intangibles to apply in what manner to what situation, such as matching the right tools (e.g. communities of practice for tacit-to-tacit exchange) to the right circumstance.
5. A dual approach of automation and human expertise for efficiency
Rather than attempting to advance either automation only or human expertise only, a more viable view is to integrate the two approaches.
A unique combination of skills and knowledge is needed to transform data into actionable decisions. Not even the most sophisticated data mining software can obviate the need for a high degree of human skill and experience in the successful analysis and use of business data. Intelligence amplification by machine and mind can outperform a mind-imitating AI system working by itself. The combination of formal, explicit knowledge in the machine, and the non-formal, tacit knowledge of the users, can thereby result in problem-solving capabilities which surpass either one of these components acting alone.
A deep understanding of how data are produced and transformed often comes only from experience. Therefore, given unusual data mining tasks or unfamiliar data mining algorithms, it is important for data mining project teams to choose team members with diverse educational backgrounds and data mining experience so that the team can make an optimal decision on choosing a data mining method.
6. The specificity of a KM task determines the effectiveness of the application of data mining techniques
To unearth possible solutions to specific challenges faced by an organisation, specific data mining techniques should be applied to specific knowledge types or a specific KM task. The consistently applied techniques identified in the reviewed articles were fuzzy logic, regression analysis, classification, artificial neural network, cluster analysis, prediction, sequential patterns, and association rules.
No one data mining algorithm has been proven to outperform other algorithms in all tasks. Therefore, in real-world data mining projects, data mining teams have to compare more than one data mining method carefully and choose one that has the best functioning performance for the KM task at hand.
The selection of data mining algorithms, hypotheses formation, and model evaluation and refinement are key components of this discovery process. Because it takes cycles of trial and error to progressively produce the most useful knowledge from data mining, a learning by experimentation approach can be useful to ensure that the process can eventually discover useful knowledge.
Header image source: Markus Spiske on Unsplash.
References:
- Peu, E. (2021). Automated Knowledge Discovery or Integration: A Systematic Review of Data Mining in Knowledge Management. (Master’s dissertation, Stellenbosch University). https://scholar.sun.ac.za/bitstream/handle/10019.1/123916/peu_automated_2021.pdf. ↩
- Moher, D., Liberati, A., Tetzlaff, J., Altman, D. G., & Prisma Group. (2009). Preferred reporting items for systematic reviews and meta-analyses: the PRISMA statement. PLoS medicine, 6(7), e1000097. ↩
Also published on Medium.