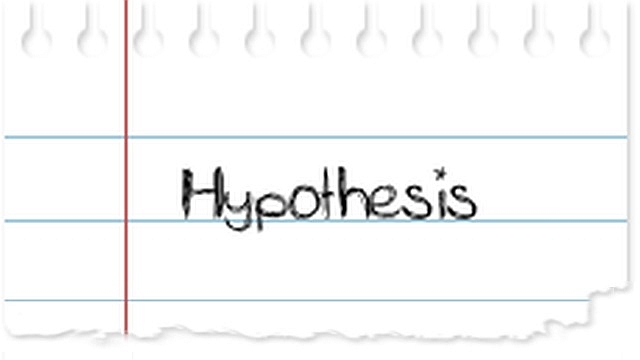
A hypothesis is a liability [Top 100 research & commentary of 2020]
This article is part 8 of a series reviewing selected papers and associated commentary from Altmetric’s list of the top 100 most discussed and shared research and commentary of 2020.
The #63 article1 in Altmetric’s top 100 list for 2020 is a September 2020 editorial in the journal Genome Biology titled “A hypothesis is a liability.” In the article, Genome Biology editors Itai Yanai and Martin Lercher argue that when researchers approach a dataset with the intent of testing a specific hypothesis, which is the focus of what they call “day science,” then the corresponding mindset may get in the way of unexpected discoveries. They contend that in this important sense, a hypothesis can be a liability, and alert that “night science” is as important as day science.
In an earlier 2019 article2 on “night science,” Yanai and Lercher advise that:
Night science is where we explore the unstructured realm of possible hypotheses, of ideas not yet fully fleshed out. In day science, we falsify hypotheses and observe which are left standing; in night science, we create them. The workings of night science are rarely discussed, as they seem abstract and less concrete compared to the logical description of the formal scientific method.
In the “A hypothesis is a liability” article, Yanai and Lercher put forward a famous selection attention experiment as part of the support for their case. If you haven’t see this experiment, it’s recommended viewing before continuing.
Before reading further, as you watch the two teams in action in following video, your task is to count the number of passes made by the team in white:
How many passes did you count? Or did you notice something else going on? Surprisingly, half of us are so focused on counting passes that we completely miss the person dressed up as a gorilla that passes through the foreground, pounding its chest with its fists. However, hardly anyone overlooks the gorilla if they haven’t been given any observation instructions before watching the video.
Wondering if a mental focus on a specific hypothesis would similarly prevent discoveries from being made when analyzing a dataset, Yanai and Lercher made up a dataset and asked students to analyze it. They described the dataset as containing the body mass index (BMI) of 1786 people, together with the number of steps each of them took on a particular day, in two files: one for men, one for women.
The students were placed into two groups. The first group was asked to consider three specific hypotheses: (i) that there is a statistically significant difference in the average number of steps taken by men and women, (ii) that there is a negative correlation between the number of steps and the BMI for women, and (iii) that this correlation is positive for men. They were also asked if there was anything else they could conclude from the dataset. In the second, “hypothesis-free,” group, students were simply asked: What do you conclude from the dataset?
The most notable “discovery” that could be made from the dataset was that if students simply plotted the number of steps versus the BMI, they would see an image of a gorilla waving at them. Yanai and Lercher advise that while students are taught the benefits of visualization, answering the specific hypothesis-driven questions did not require plotting the data. They found that very often, the students driven by specific hypotheses skipped this simple step towards a broader exploration of the data. In fact, overall, students without a specific hypothesis were almost five times more likely to discover the gorilla when analyzing this dataset.
In concluding their “A hypothesis is a liability” editorial, Yanai and Lercher advise researchers to:
…keep your mind open when working with data. Think about the particular dimensionality of your dataset and study the variation across these. Consider what the variation along these dimensions may reflect, and try to connect that to aspects beyond the dataset. By asking what other dimensions could be integrated to explain the observed variation, you are positioning yourself for a discovery. Let your fantasies run wild to generate classes of hypotheses that would leave traces in the data. There could be gorillas hiding in there.
Criticism of “A hypothesis is a liability”
In February 2021 correspondence3 in Genome Biology, Teppo Felin, Jan Koenderink, Joachim I. Krueger, Denis Noble & George F.R. Ellis disagree with the claims made by Yanai and Lercher in their “A hypothesis is a liability” editorial.
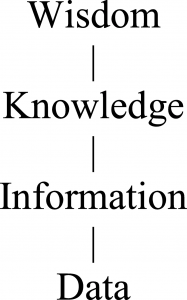
In their correspondence, titled “The data-hypothesis relationship,” Felin and colleagues argue that while they agree that a bad hypothesis is a liability, they consider that there is no such thing as hypothesis-free data exploration. They state that observation and data are always hypothesis- or theory-laden, and that any exploration of data, however informal, will necessarily be guided by some form of expectations. They contend that even informal hunches or conjectures are types of proto-hypothesis. In consideration of this, Felin and colleagues argue that hypothesis-free observation is neither possible or desirable, and that hypotheses are actually the primary engine of scientific creativity and discovery.
As part of the support for their argument, Felin and colleagues contend that Yanai and Lercher have incorrectly specified the data-hypothesis relationship by privileging the role of the data to the detriment of hypothesis and theory. In their correspondence, Felin and colleagues use the DIKW hierarchy from knowledge management (KM) to illustrate this point, as shown in Figure 1. They state that the currently popular data-first approaches assume that scientific understanding is built from the bottom-up. However, they argue that to the contrary, many of the greatest insights have come “top-down,” where scientists start with theories and hypotheses that guide them to identify the right data and evidence.
Yanai and Lercher’s response to the criticism
In a February 2021 reply4 to the criticism of Felin and colleagues titled “The data-hypothesis conversation,” Yanai and Lercher argue that Felin and colleagues have invalidated their criticism by inappropriately changing the very definition of the term “hypothesis,” making it absurdly broad. Yanai and Lercher state that with this redefinition, “hypothesis” becomes a synonym for mental constructs, meaning that no conscious human activity can ever be hypothesis-free, but argue that this thinking completely misses the point of what hypotheses mean to science.
Further, Yanai and Lercher also advise that contrary to the linear top-down/bottom-up DIKW model put forward by Felin and colleagues, research projects actually follow a convoluted progression as shown in Figure 2. While a question or hypothesis drives each collection of data, each new question or hypothesis is in turn triggered by the analysis of an earlier dataset.
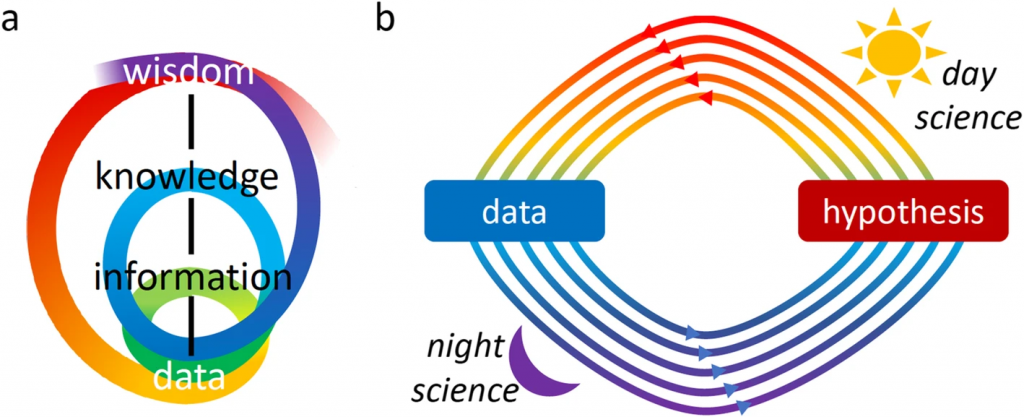
What does this mean for knowledge management?
We need both “day science” and “night science”
What Yanai and Lercher refer to as “day science” – where hypotheses are formulated and rigorously tested – is a vital and indispensable aspect of evidence-based KM.
However, so is what they refer to as “night science.” For example, it allows us to access unconscious knowledge and understanding, and helps us to identify hidden patterns during the sensemaking step of knowledge strategy processes (and indeed, the gorilla video activity above had been included in this particular sensemaking step to highlight the benefits of what Yanai and Lercher refer to as “night science”).
So both “day science” and “night science” are needed, and Yanai and Lercher support this in their February 2021 reply, where they state that:
While we wrote about night science and hypothesis-free explorations, we gladly admit that hypotheses occupy a central space in day science. Any hypothesis … must be subjected to rigorous attempts at falsification, and this is clearly the domain of day science. What we mean to suggest is that the hypothesis-testing part is only half of the process; the other half, comprising the untold story of how hypotheses are generated, deserves the same attention. Science owes much of its progress to serendipity—to unexpected, unplanned findings. Data exploration beyond specific hypotheses may increase our chances to stumble upon such serendipitous discoveries.
Shortcomings of the DIKW model
The concerns raised by Yanai and Lercher in regard to the oversimplified and linear nature of the DIKW model are shared by others5.
As he discusses in RealKM Magazine, David Williams has put forward the Action-Knowledge-Information (AKI) model6 shown in Figure 3 as a better representation of the relationships between data, information, knowledge (and wisdom).
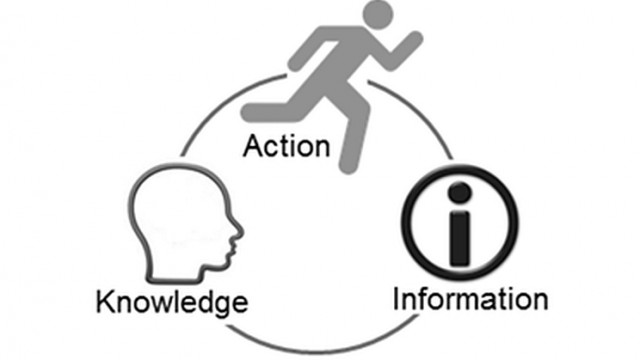
References:
- Yanai I, & Lercher M. (2020). A hypothesis is a liability. Genome Biology, 21, 231. ↩
- Yanai, I., & Lercher, M. (2019). Night science. Genome Biology, 20, 179. ↩
- Felin, T., Koenderink, J., Krueger, J. I., Noble, D., & Ellis, G. F. (2021). The data-hypothesis relationship. Genome Biology, 22, 57. ↩
- Yanai, I., & Lercher, M. (2021). The data-hypothesis conversation. Genome Biology, 22, 58. ↩
- Intezari, A., Pauleen, D. J., & Taskin, N. (2016, January). The DIKW hierarchy and management decision-making. In 2016 49th Hawaii International Conference on System Sciences (HICSS) (pp. 4193-4201). IEEE. ↩
- Williams, D. (2014). Models, metaphors and symbols for information and knowledge systems. Journal of Entrepreneurship, Management and Innovation, 10(1), 80-109. ↩
Also published on Medium.