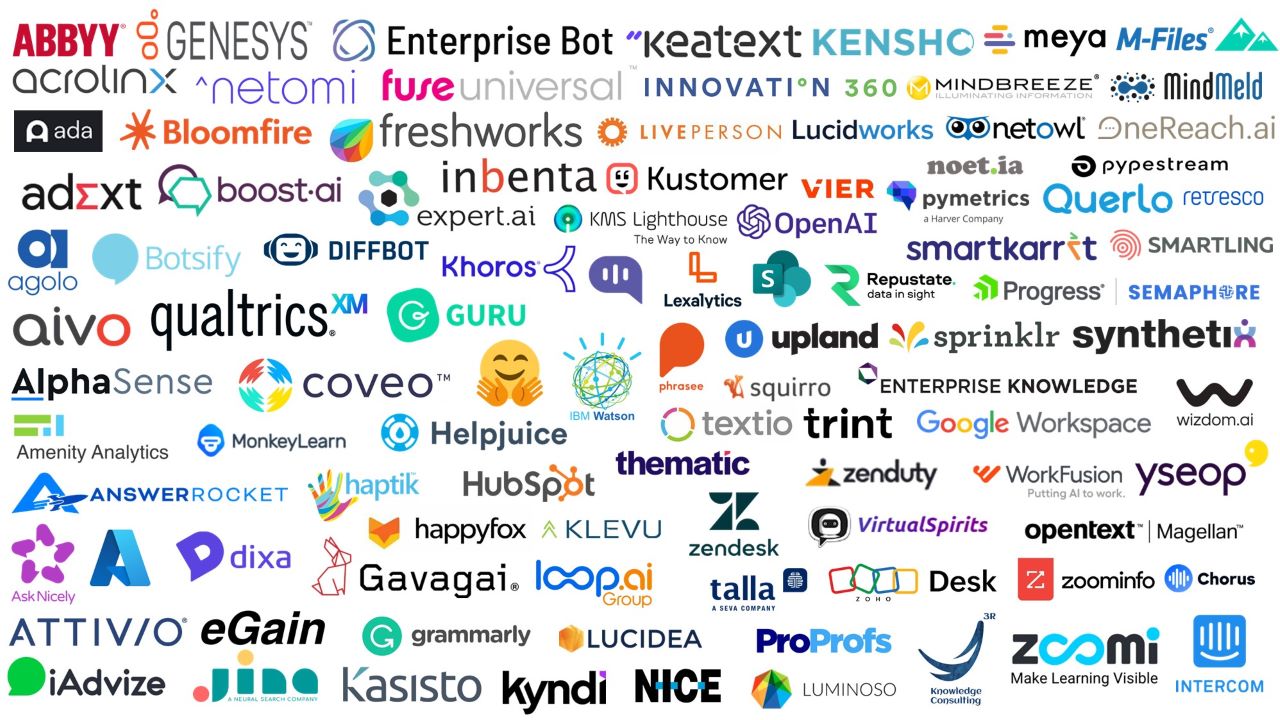
AI-based KM features for knowledge discovery and generation [Generative AI & KM series part 6]
This article is part 6 of the series AI integration strategy for learning and knowledge management solutions.
A comparative study of 100 generative AI tools in the context of learning and knowledge management (KM) was conducted and has resulted in a set of 35 KM processes where generative AI1 has augmented their experience, implementation, and execution. This article (part 6 in the series) focuses on knowledge discovery and generation KM processes.
Part 6. Knowledge discovery & generation
6.1. Content views / topic-based dashboard
With the help of generative AI, emerging topics are showed in customized dashboard view based on what an audience groups are searching for. Views are created based on topics that represent stakeholders’ interests and expected information/ knowledge. Views can also be created based on the role (Level 1, Level 2, etc.) the user can view appropriate levels of information while using different methods to access the knowledge base. AI algorithms can suggest the creation of topical pages to expand digital reach and meet new audience needs and interests. Domain experts can find information and documents specific to their line of work, whether it’s sales, legal, or engineering. AI can personalize the employees’ personal space in a knowledge base with dynamic content suggestions and tailor content based on employee role, activity, topics of interests or specific views. Views can also feature content which is often used to promote time-sensitive communication to increase visibility and reachability.
6.2. Content optimization (accuracy, clarity…)
With the help of generative AI, content goals can be set for content optimization. A goal is a content characteristic which the AI algorithm is going to check the content for. In other words, content goal is a set of guidance that fits the content strategy and helps the contributors/ writers stay aligned. For example, a global-ready content will likely be translated and localized, so content goals such as clarity, consistency, and scan-ability (for easy readability) are important goals. More example on content goals can be insights on gendered phrases, harmful language, age bias, corporate clichés, engaging phrases, brand keywords, fixed mindset language.
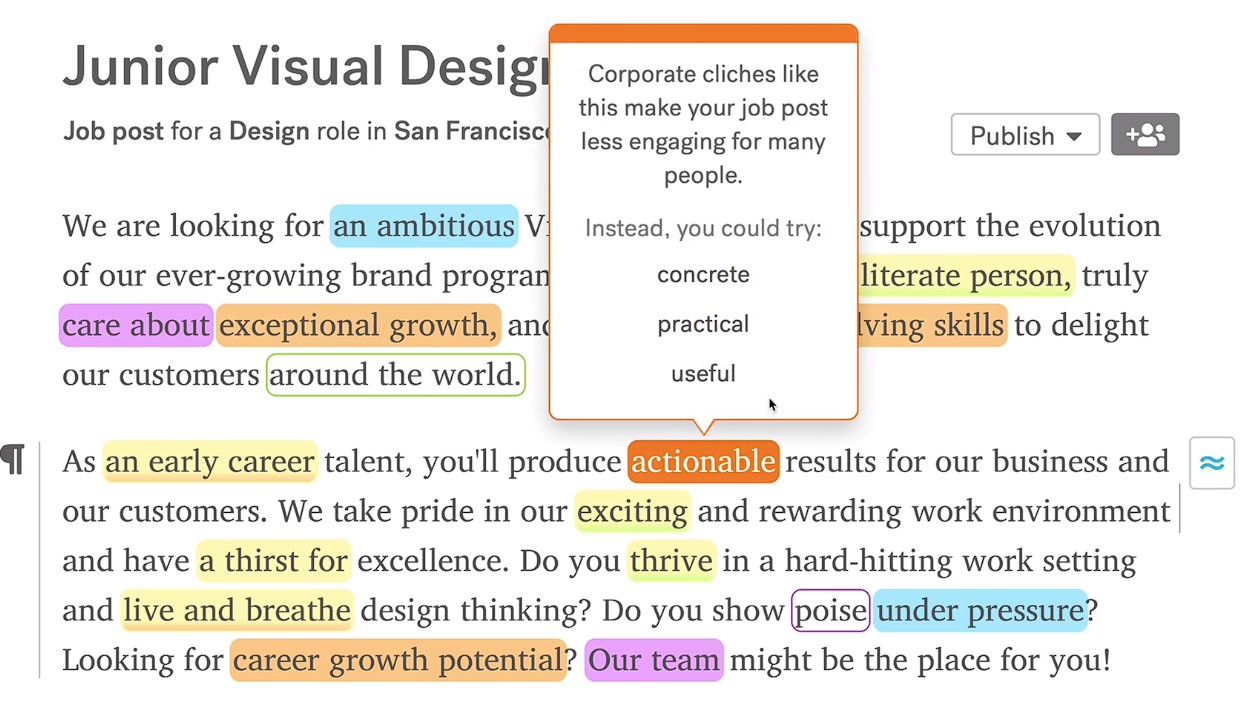
AI content optimization algorithms can also measure behaviors and track them over time. For example, a change leader may need to quantify the impact of the inclusion efforts by tracking changes in the entire team’s language over time.
6.3. Content automation & operational efficiency
With the help of generative AI, process managers can build complex workflows and connect them with core systems. As result the AI application is not limited to responding to queries but also take action to resolve them. With the human supervision the AI algorithm can map out the end-to-end processes and know exactly where to step in and deploy content automation modules that support the front-end people and improve their resolution experience with customers. Internally, employees can request services in a centralized interface for any function ranging from IT, HR to finance, facilities, and other lines of business. AI features can make processes to flow seamlessly with a simple drag and drop workflow builder, allowing users to collaborate efficiently with cross-functional teams, and effortlessly scale the operations for efficient resolution of customer inquiries.
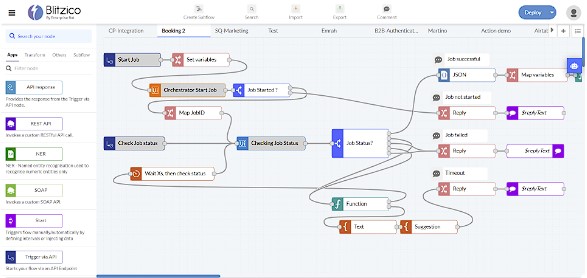
6.4. Recommend relevant/confident content
With the help of generative AI and using self-organizing techniques, the knowledge application offers relevant answers to customer requests based on dynamic relevance ranking and human supervision. The most relevant contents are surfaced in one list, regardless of format or where they reside – in specific applications, websites, internal servers, community discussions, blogs, wikis, library and more.
Search results also include federated & unstructured knowledge to provide highly relevant results which increase knowledge re-discovery. An example, in the context of problem solving, a community site may deploy ‘critical knowledge finder’ an application that returns relevant answers that qualify as possible solutions. It returns also similar and related problems for further discovery of potential solutions in case of variations in the problem conditions and context.
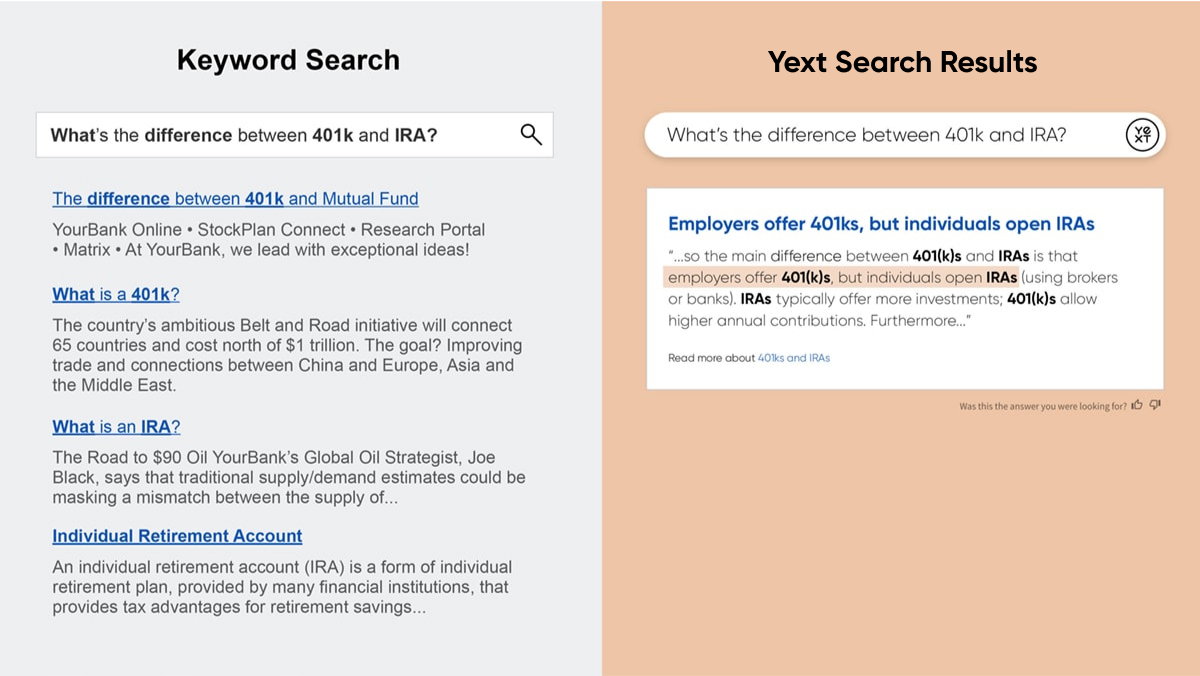
The recommendation engine goes beyond keyword matching. It delivers specific, relevant answers by comprehending the context and intent behind queries. With answers rooted in trusted enterprise content, users can trace back the origins of the returned answers, fostering a deeper understanding and trust in the results. The recommendation engine also builds semantic relationships that are established between different data sources and data from one source can be used to enrich data in another source. As a result, the recommendation engine can identify and extract correlations and dependencies between the existing information and provide relevant results on a priority basis.
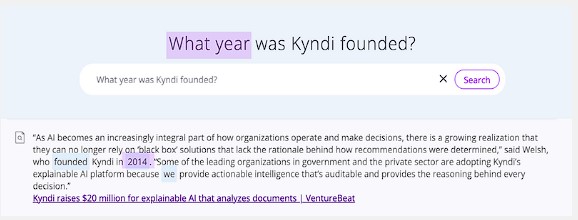
6.5. Content re-generation & conversational search
With the help of generative AI and using machine learning techniques content re-generation or content extraction into a new format or schema can be simplified and/or automated. For example, content from websites and their spider links, articles, product pages, discussions can be automatically extracted without any business rules for content retrieval. For a webpage, content is interpreted by a machine learning model trained to identify the key attributes on a page based on its type. The result is a website transformed into clean structured data (like JSON or CSV), ready to be imported into business application. Content regeneration can act on specific sections of a long report and regenerate it into a new document based on pre-built questions in specific domain areas. It supports content extraction from PDFs and even extracting content within images using advanced OCR techniques.

Content re-generation using smart document understanding (SDU) enables users to label text so that the tool builds an understanding of critical components inside the enterprise documentation, such as headers, tables and more. With the human supervision, an initial annotation of few documents’ pages, the SDU can teach itself the rest, retrieving answers and information only from relevant content. It transforms unstructured documents into a machine-readable format that organizes the headers, titles, paragraphs, tables, and footers detected within the document in natural reading order. AI algorithms interpret messy page layouts, structuring text into cohesive paragraphs that can then be effectively analyzed and searched.
In conversational search, users may engage in conversations to get educated on a given topic, to solve problems, to troubleshoot an issue or simply feed their curiosity. AI conversational search algorithms examine long-form unstructured documents (like blogs, bios, support articles, product manuals) and returns search results based on the user prompt, intent, and context. Users engage in free flowing & personalized conversations by retaining the context of users’ previous messages. Meta-data are added in the process of text extraction. For example, a new hired engineer may engage in a conversational search to better understand key concepts on designing a wind turbine. The AI search algorithms will offer quick access to technical information that is stored in various external data sources and document formats to answer the questions of the newly hired engineer. Conversational search can also recommend questions to newly hires to start with then they can easily follow up on their own and lower the barrier to an effective onboarding experience.
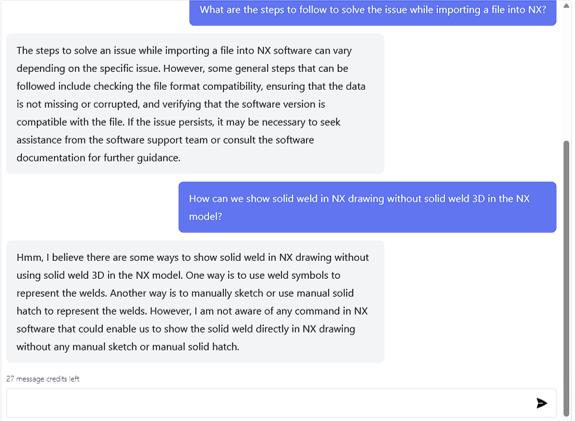
Conversational search may also be applied to transform boring web forms into friendly conversations, in this way information from abandoned forms are collected for further analysis or follow up with the customer. Conversational search features predictive query suggestions and typo tolerance to ensure employees find the most accurate, relevant, and useful content based on intent rather than exact keyword match. Conversational search can be coupled with cognitive based search on the question, so it finds the most important paragraph of the documents where the topic is explained. It can also be tied with semantic search, so if the user searches for Bank, he/ she will get loan, credit, finance in the results as well.
Examples of AI-based KM tools for knowledge discovery and generation: Textio, Mindbreeze, 3R Knowledge, Kyndi, DiffBot, Enterprise Bot, Yext.
Next part (part 7): AI-based KM features for knowledge-centered services.
Header image source: Author provided.
Reference:
- Najjar, R. (2023, July 13). Preliminary Understanding of Generative AI: What & How? Medium. ↩