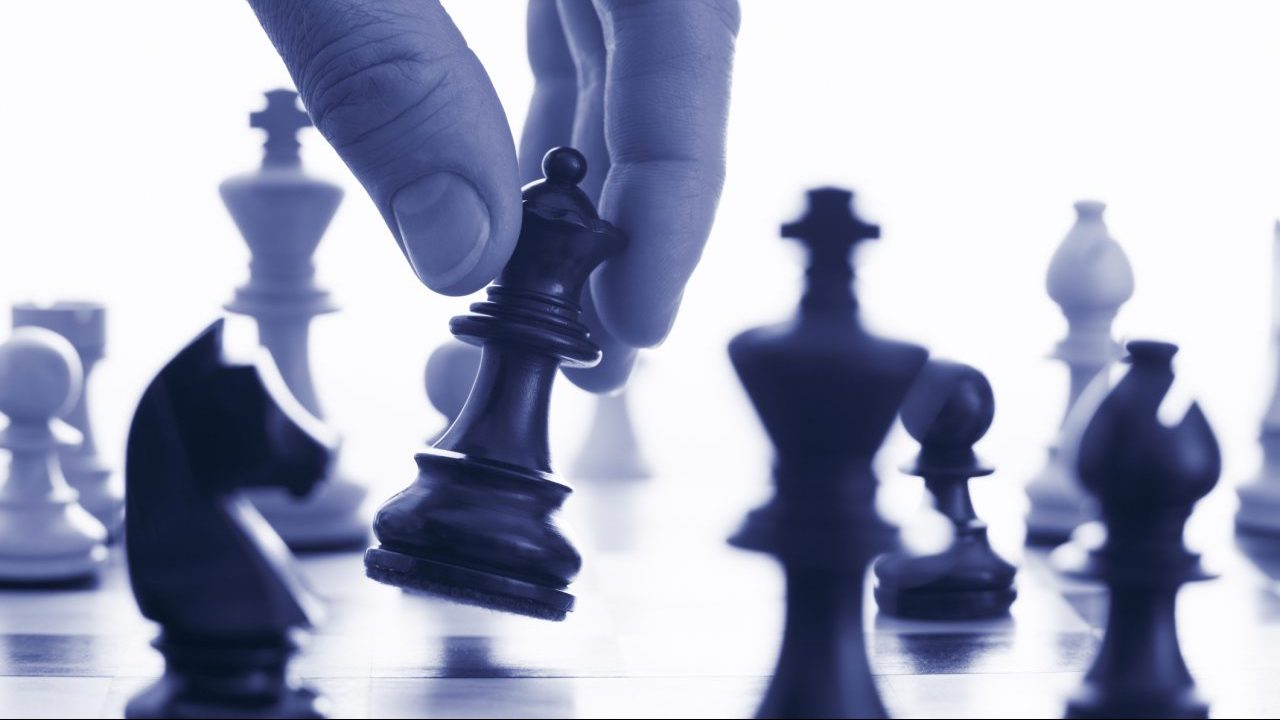
Why men and machine are the best team in materials science
Originally posted on The Horizons Tracker.
The last few years have seen a deluge of articles enthusiastically proclaiming the latest technological breakthrough. These breakthroughs are often accompanied by concerns about how humans will cope in the face of their new technological overlords.
It’s a narrative that former chess grandmaster Garry Kasparov knows only too well after his famous battle with IBM’s Deep Blue in the 1990s, when his defeat prompted many to proclaim the age of machines to have begun. Kasparov takes a slightly more measured perspective on matters, and argues that “weak human + machine + better process” is nearly always better than a machine acting alone.
It’s an approach that was highlighted in a recent study1 conducted at the Oak Ridge National Laboratory into the potential of man and machine to work effectively together in the field of materials science.
Material gain
Materials science is a discipline with a natural leaning towards big data and machine learning, as initiatives such as the Materials Genome Initiative have seen vast quantities of data about the materials we know and use today. It’s understandable to expect machine learning to make sense of this data and propose fundamentally new designs of next-generation materials. Indeed, technology can perform the trial-and-error that has remained the bedrock of human discovery on an industrial scale.
The realm of complex materials can be too much even for the most sophisticated computers however, and the research explains how combining the abilities of man and machine can crack some of the hardest problems in the field today.
The researchers achieve this via an approach known as dimensional stacking, which involves human knowledge helping to intelligently organize the data that the machines have to then analyze.
“When your machine accepts strings of data, it really does matter how you are putting those strings together,” the researchers explain. “We must be mindful that the organization of data before it goes to the algorithm makes a difference. If you don’t plug the information in correctly, you will get a result that isn’t necessarily correlated with the reality of the physics and chemistry that govern the materials.”
Preprocessing data
As such, the researchers propose a process whereby the data is organised and prepared before being fed into the system. The organisation is typically done along the physical and chemical properties of the material that are most of interest to material scientists themselves.
The researchers believe that scientists have a decent idea about the potential for physical or chemical correlations, and this can help to organise and structure the data in a way that helps the algorithms to make sense of it.
The method was put through its paces with a sample of data on relaxor-ferroelectric materials, which are commonly used in advanced ultrasonic imaging equipment. In total, the researchers developed hundreds of thousands of data points to allow for robust testing of the approach, and they discovered a number of new things about relaxor-ferroelectric materials, including that they have a disorderly behaviour that’s reminiscent of materials such as glass, which is something that was not widely known beforehand.
Man and machine in the workplace
It’s a finding that has clear implications for the field of materials science, but what about other forms of work environment? Research from Accenture Strategy provides a reminder of just how wide this potency spreads.
They assessed the impact of technology such as artificial intelligence on the workplace from the perspective of how organisations can best make use of it. They suggest that employers should be investing heavily in skills development so that employees have the skills required to work effectively with the new technologies entering the workplace.
In many instances, these skills will include human, soft skills such as creativity, leadership, critical thinking and emotional intelligence, with those organisations that invest in these skills likely to reap the rewards in terms of successful digital transformation. What’s more, it also buffers the impact of technology in terms of job displacement, with Accenture suggesting redundancies would half if this kind of skills investment is made.
“Paradoxically, the truly human skills, from leadership to creativity, will remain highly relevant and winning organizations will strike the right balance – leveraging the best of technology to elevate, not eliminate their people,” Accenture say. “Not only are workers optimistic, but they understand they must learn new skills. Digital can accelerate learning by embedding training seamlessly into daily work – so learning becomes a way of life – helping workers and organizations remain relevant.”
They propose a number of steps that can be taken to ensure employees have the skills needed to work effectively with new technologies:
- Investing in training – An obvious first step is to prioritise training by increasing investment in it. This investment should cross both technical and soft skills.
- Redesign work – New ways of working, and even new roles entirely, are likely as a result of new technologies in the workplace, so a degree of process re-engineering is inevitable.
- Develop a talent pipeline – Most industries are complaining about talent shortages, so it’s imperative that companies take responsibility and start investing in their workforce so that these problems don’t affect them.
It’s clear that Kasparov’s Law on AI does have significant merit, but it’s also true that a lot of work is required to ensure that people have the skills required to work effectively alongside AI and other new technologies. There’s no better time to start developing those skills than now.
Article source: Why Men And Machine Are The Best Team In Materials Science.
Reference:
- Griffin, L. A., Gaponenko, I., Zhang, S., & Bassiri-Gharb, N. (2019). Smart machine learning or discovering meaningful physical and chemical contributions through dimensional stacking. npj Computational Materials, 5(1), 1-8. ↩