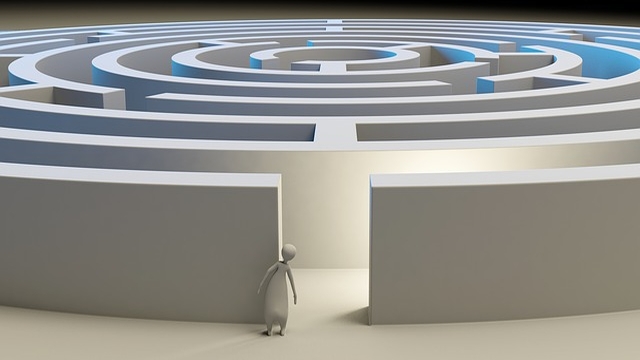
Exploring the science of complexity series (part 13): Concept 4 – Nonlinearity
This article is part 13 of a series of articles featuring the ODI Working Paper Exploring the science of complexity: Ideas and implications for development and humanitarian efforts.
Complexity and systems – Concepts 4, 5, 6, and 7
The next four concepts relate to different aspects of how complex systems – those characterised by Concepts 1-3 – change over time. The causal relationships that play out within complex systems are explained using the concept of nonlinearity (Concept 4) and the sensitivity of complex systems to their starting conditions is highlighted (Concept 5). The overall shape of the system and its future possibilities are described using the idea of phase space (Concept 6). The patterns underlying seeming chaos within complex systems are explained (Concept 7).
Concept 4 – Nonlinearity1
… the darkest corner of science [is] the realm of non-linear problems2
Outline of the concept
Traditional scientific approaches are based on the idea that linear relationships can be identified through data gathering and analysis, and can be used as the basis of ‘laws’ of behaviour3. Such approaches in the physical sciences have informed the development of social, economic and political science, using broad theories of behaviour to generate hypotheses about causal relations between variables of interest4. However, complexity science suggests that human systems do not work in a simple linear fashion. Feedback processes between interconnected elements and dimensions lead to relationships that see change that is dynamic, nonlinear and unpredictable5. Nonlinearity is a direct result of the mutual interdependence between dimensions found in complex systems. In such systems, clear causal relations cannot be traced because of multiple influences.
The distinction between linear and nonlinearity is far from trivial. If dynamic nonlinear feedbacks in response to rising greenhouse gases are included in the model used in the Stern Review of Climate Change (cited in Concept 2), for example, the total average cost of climate change rises from 5% to at least 20% of global per capita consumption6 7.
Detailed explanation
Vast numbers of naturally occurring systems exhibit nonlinearity. As one thinker has dryly suggested (Stanislaw Ulam, in the 1950s), calling a situation nonlinear is like going to the zoo and talking about all the interesting non-elephant animals you can see there8: there are as many nonlinear situations as there are non-elephant animals.
Linearity describes the proportionality assumed in idealised situations where responses are proportional to forces and causes are proportional to effects9. Linear problems can be broken down into pieces, with each piece analysed separately; finally, all the separate answers can be recombined to give the right answer to the original problem. In a linear system, the whole is exactly equivalent to the sum of the parts. However, linearity is often an approximation of a more complicated reality – most systems only behave linearly if they are close to equilibrium and are not pushed too hard. When a system starts to behave in a nonlinear fashion, ‘all bets are off’ 10.
This is not to suggest that nonlinearity is necessarily a dangerous or unwanted aspect of systems. The biology of life itself is dependent on nonlinearity, as are the laws of ecology. Combination therapy for HIV/AIDS using a cocktail of three drugs works precisely because the immune response and viral dynamics are nonlinear – the three drugs taken in combination are much more effective than the sum of the three taken separately.
The nonlinearity concept means that linear assumptions of how social phenomena play out should be questioned. It is important to note that such thinking has only relatively recently been incorporated into the ‘hard’ science paradigms and, moreover, is still only starting to shape thinking in the social, economic and political realms. Nonlinearity poses challenges to analysis precisely because such relationships cannot be taken apart – they have to be examined all at once, as a coherent entity. However, the need to develop such ways of thinking cannot be overstated – as one thinker puts it:
… every major unresolved problem in science – from consciousness to cancer to the collective craziness of the economy, is nonlinear11
Although nonlinearity is a mathematical formulation, it is useful to take the suggestion that what is required is a ‘qualitative understanding of [the] quantitative’ when attempting to investigate them systematically12. Such a qualitative understanding has been furthered by the work of Robert Jervis13 on the role of complexity in international relations. Starting with the notion that understanding of social systems has tacitly incorporated linear approaches from Newtonian sciences, Jervis goes on to highlight three common assumptions that need to be challenged in order to take better account of nonlinearity. These assumptions provide a solid basis for investigating nonlinearity.
First, it is very common to test ideas and propositions by making comparisons between two situations which are identical except for one variable – referred to as the independent variable. This kind of analysis is usually prefaced with the statement ‘holding all other things constant’. However, in a system of interconnected and interrelated parts, with feedback loops, adaptive agents and emergent properties, this is almost impossible, as everything else cannot be held constant and there is no independent variable. Jervis argues that, in such systems, it is impossible to look at ‘just one thing’, or to make only one change, hence to look at a situation involving just one change is unrealistic.
Secondly, it is often assumed that changes in system output are proportional to changes in input. For example, if it has been assumed that a little foreign aid slightly increases economic growth, then more aid should produce more growth. However, as recent work by ODI and others argues, absorption capacity needs to be taken account – more aid does not necessarily equate to better aid. In complex systems, then, the output is not proportional to the input. Feedback loops and adaptive behaviours and emergent dynamics within the system may mean that the relationship between input and output is a nonlinear one:
Sometimes even a small amount of the variable can do a great deal of work and then the law of diminishing returns sets in [a negative feedback process] … in other cases very little impact is felt until a critical mass is assembled14
The third and final commonly made assumption of linearity is that the system output that follows from the sum of two different inputs is equal to the sum of the outputs arising from the individual inputs.
In other words, the assumption is that if Action A leads to Consequence X and Action B has Consequence Y then Action A plus Action B will have Consequences X plus Y.
This frequently does not hold, because the consequences of Action A may depend on the presence or absence of many other factors which may well be affected by B or B’s Consequence (Y). In addition, the sequence in which actions are undertaken may affect the outcome.
Example: The growth dynamics model as an alternative to linear regression models
Studies of economic growth face methodological problems, the foremost of which is dealing with real world complexity. The standard way of understanding growth assumes, implicitly, that the same model of growth is true for all countries, and that linear relationships of growth are true for all countries. However, linear relationships might not apply in many cases. An example would be a country where moderate trade protection would increase economic growth but closing off the economy completely to international trade would spell economic disaster. Linear growth models imply that the effect of increasing the value of the independent variable would be the same for all countries, regardless of the initial value of that variable or other variables. Therefore, an increase of the tariff rate from 0% to 10% is presumed to generate the same change in the growth rate as a change from 90% to 100%. Furthermore, the change from 0% to 10% is assumed to have the same effect in a poor country as in a rich country, in a primary resource exporter as in a manufacturing exporter, and in a country with well developed institutions as in a country with underdeveloped institutions. Despite some efforts to address these issues by relaxing the linear framework and introducing mechanisms to capture nonlinearities and interactions among some variables, this is still a poor way of addressing real world nonlinearity. Econometric research has identified that linear models cannot generally be expected to provide a good approximation of an unknown nonlinear function, and in some cases can lead to serious misestimates15.
Research at Harvard University has focused on the problem of designing a growth strategy in a context of ‘radical uncertainty’ about any generalised growth models. They call their method ‘growth diagnostics’, in part because it is very similar to the approach taken by medical specialists in identifying the causes of ailments. In such a context, assuming that every country has the same problem is unlikely to be very helpful. The principal idea is to look for clues in the country’s concrete environment about the specific binding constraints on growth. The growth diagnostics exercise asks a set of basic questions that can sequentially rule out possible explanations of the problem. The answers are inherently country-specific and time-specific. The essential method is to identify the key problem to be addressed as the signals that the economy would provide if a particular constraint were the cause of that problem.
Implication: Challenge linearity in underlying assumptions
Within complex systems, the degree of nonlinearity and relationships between various factors, and the lack of proportionality between inputs and outputs, means that the dynamics of change are highly context-specific. Therefore, if there are assumptions, aggregations and theories about the relations among different aspects of a specific situation, and these are not entirely appropriate when applied to the dynamics of a new local situation, then this perspective is unlikely to lead to a deep understanding of what should be done, and is furthermore unlikely to lead to the hoped-for changes.
Nonlinearity implies that, as well as understanding the limitations of a particular model or perspective, it is important to build and improve new models that can provide the sort of information required for the particular task at hand.
No kind of explanatory representation can suit all kinds of phenomena … any one diagnosis of [a] problem and its solution is necessarily partial16
From this perspective, it is important to tailor to the particular situation one’s perspective on the dynamics of some phenomena. In a complex system, one must examine the complex web of interrelationships and interdependencies among its parts or elements17. It is important from the outset to understand the association and interaction among variables, rather than assuming that one causes another to change, and to look at how variables interact and feed back into each other over time18. Homer-Dixon, cited above, suggests that political scientists use methods that are modelled on the physical sciences, developing broad theories of political behaviour to generate hypotheses about causal relations between variables of interest.
These ideas resonate strongly with a recent assessment undertaken for Sida on the use of the log frame19, highlighting some of the advantages and disadvantages in a way which is particularly pertinent for this paper. In the international aid world, much of programme planning and development is undertaken using a set of methods and tools called the logical framework. For most of the study respondents, the advantage of logical frameworks was that they force people to think carefully through what they are planning to do, and to consider in a systematic fashion how proposed activities might contribute to the desired goal through delivering outputs and outcomes. As a result, many see the log frame as a useful way of encouraging clear thinking.
However, these positive aspects were offset by the almost universal complaint that the log frame rests on a very linear logic, which suggests that if Activity A is done, Output B will result, leading to Outcome C and Impact D. This linear idea of cause and effect is profoundly ill-at-ease with the implications of complexity science and, indeed, the experiences of many development practitioners. The authors of the study sum up the problems of the log frame in a way that is key to our discussion of complexity:
Unfortunately (for the logical framework approach at least) we are not working with such a self-contained system and there are so many factors involved which lie beyond the scope of the planned initiative that will change the way things work. Although the LFA makes some attempt to capture these through the consideration of the risks and assumptions, these are limited by the imagination and experience of those involved. As a result the LFA tends to be one-dimensional and fails to reflect the messy realities facing development actors20
Nonlinearity also has clear implications for the increased interest in randomised control trials (RCTs). While the implications of nonlinearity for techniques and tools such as the log frame and RCTs are increasingly well understood by many actors within the aid system, the answer to the deeper question as to whether incorporation of nonlinearity will be feasible, given the pressure on donors to justify aid budgets while having to deal with a reducing headcount, is less clear. The distinction between linearity and nonlinearity can be seen in as providing a theoretical underpinning of the frequently cited tension between upward accountability and learning. It also provides a means to re-frame the debate. If the two goals of accountability and learning are also about different mindsets, the degree to which an appropriate balance can be struck – without exploring these mindsets and the assumptions on which they are based – is open to question.
Next part (part 14): Concept 5 – Sensitivity to initial conditions.
Article source: Ramalingam, B., Jones, H., Reba, T., & Young, J. (2008). Exploring the science of complexity: Ideas and implications for development and humanitarian efforts (Vol. 285). London: ODI. (https://www.odi.org/publications/583-exploring-science-complexity-ideas-and-implications-development-and-humanitarian-efforts). Republished under CC BY-NC-ND 4.0 in accordance with the Terms and conditions of the ODI website.
Header image source: qimono on Pixabay, Public Domain.
References and notes:
- It is important to distinguish nonlinearity as used here, which relates to relationships and proportionality, and nonlinearity in terms of sequences of events – one thing following another. ↩
- Strogatz, S. (2003). Sync: The Emerging Science of Spontaneous Order, London: Penguin Books. ↩
- Byrne, D. (1998). Complexity Theory and the Social Sciences: An Introduction, London: Routledge. ↩
- Homer-Dixon, T (1995). Strategies for Studying Causation in Complex Ecological Political Systems, Occasional Paper Project on Environment, Population and Security Washington, D.C.: American Association for the Advancement of Science and the University of Toronto. ↩
- Stacey, R. (1996). Complexity and Creativity in Organisations, San Francisco: Berrett-Koehler Publishers. Stanford Encyclopaedia of Philosophy (2006) ‘Emergent Properties’. ↩
- HM Treasury (2006). The Stern Review of the Economics of Climate Change, London: HM Treasury. ↩
- Note that the previously cited increase from 5 to 14.4% was due to natural, known feedbacks and does not include non-linear feedbacks. ↩
- Campbell, D., Farmer, D., Crutchfield, J. and Jen, E. (1985). ‘Experimental Mathematics: The Role of Computation in Nonlinear Science’, Communications of the Association for Computing Machinery 28(4):374–84. ↩
- Strogatz, S. (2003). Sync: The Emerging Science of Spontaneous Order, London: Penguin Books. ↩
- Strogatz, S. (2003). Sync: The Emerging Science of Spontaneous Order, London: Penguin Books. ↩
- Capra, F. (1996). The Web of Life, London: Flamingo/Harper Collins. ↩
- Byrne, D. (1998). Complexity Theory and the Social Sciences: An Introduction, London: Routledge. ↩
- Jervis, R. (1997). System Effects: Complexity in Political and Social Life, Princeton, NJ: Princeton University Press. ↩
- Jervis, R. (1997). System Effects: Complexity in Political and Social Life, Princeton, NJ: Princeton University Press. ↩
- Rodríguez, F (2007). Policymakers Beware: The Use and Misuse of Regressions in Explaining Economic Growth, Policy Research Brief No. 5, International Poverty Centre, Brasilia: IPC. ↩
- Holland, J. (2000). Emergence: From Chaos to Order, Oxford: OUP. ↩
- Flynn Research (2003). Complexity Science: A Conceptual Framework for Making Connections, Denver: Flynn Research. ↩
- Haynes, P. (2003). Managing Complexity in the Public Services, Berkshire: Open University Press. ↩
- Bakewell, O. and Garbutt, A. (2005). The Use And Abuse Of The Logical Framework Approach, Stockholm: Sida. ↩
- Bakewell, O. and Garbutt, A. (2005). The Use And Abuse Of The Logical Framework Approach, Stockholm: Sida. ↩