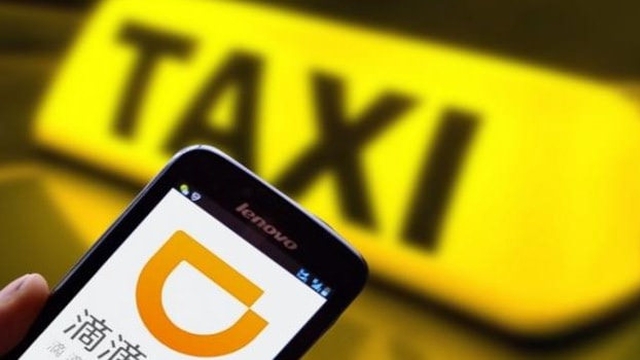
How AI can lead to smarter cities
Originally posted on The Horizons Tracker.
Much of the ‘management’ side of companies such as Uber is done by algorithms that determine pricing and distribution of drivers on behalf of passengers. A recent study1 suggests that AI can be used to predict the demand for taxi and ride sharing services as a whole, leading to safer, smarter and more sustainable cities.
The paper documents the creation of two types of neural networks that were set to work analyzing the demand for taxis in a city. Once the algorithms had been trained on this data, they were able to accurately predict future demand patterns with a higher degree of accuracy than existing technology.
“Ride sharing companies, like Uber in the United States, and Didi Chuxing in China, are becoming more and more popular and have really changed the way people approach transportation,” the authors say. “And you can imagine how important it would be to predict the taxi demand because the taxi company could dispatch the cars even before the need arises.”
Smarter transportation
The team analyzed data of ride requests from the Chinese taxi company Didi Chuxing. These requests, which are usually made via an app, provides researchers with an accurate reflection of demand because not all requests are fulfilled.
“This is really good data because it’s based on demand,” the authors say. “If you just know how many people took a ride, that doesn’t really tell you the demand because it could be that people didn’t get a ride, or others just gave up trying.”
This data, which usually includes the time and location of each request, allows researchers to build up a detailed overview of how demand changed over time. This was then visualized on a map to allow them to see the demand evolve, both over the course of the day but also over longer time periods.
“In the morning, for example, you can see that in a residential section there are more pickups, and there are more drop-offs in the downtown area,” they explain. “In the evening, it’s reversed. What we are doing is using historical pickup data to predict how this map changes 30 minutes from now, one hour from now, and so on.”
As with so many AI-driven projects, this one benefited tremendously from access to very large data sets and high-powered computer technology to process this huge quantity of data. Whilst the project has yet to develop anything for the market, it will be fascinating to see what the team’s next steps are.
Article source: How AI Can Lead to Smarter Cities.
Header image source: Adapted from Apple invierte 1000M en el Uber chino para potenciar el Apple Car by iphonedigital which is licenced by CC BY-SA 2.0.
Reference:
- Yao, H., Wu, F., Ke, J., Tang, X., Jia, Y., Lu, S., … & Ye, J. (2018). Deep multi-view spatial-temporal network for taxi demand prediction. arXiv preprint arXiv:1802.08714. ↩