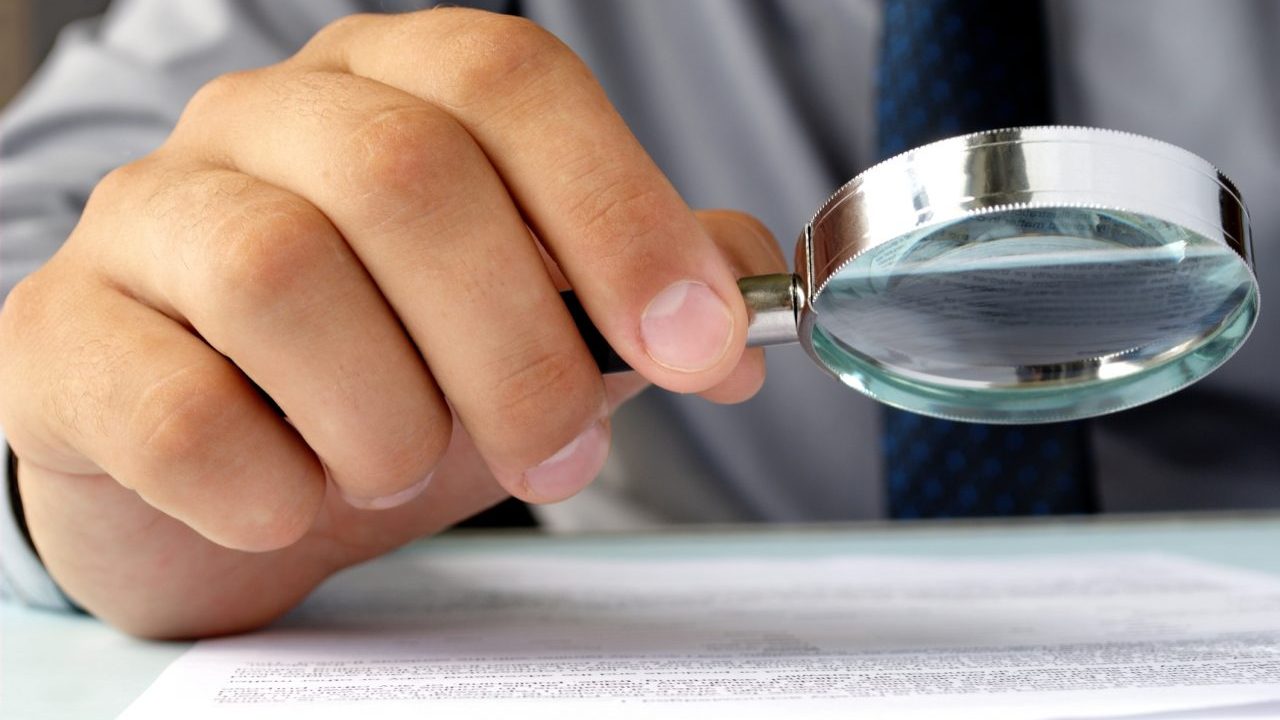
What causes bias in scientific literature?
Increasing scrutiny is being focused on several serious problems in science, including researchers being unable to reproduce the findings of prominent studies, statistical significance being misused and misinterpreted, the hyping of study findings, scientific fraud, and researchers publishing in low-quality predatory journals.
A range of biases are believed to be behind these problems, but their prevalence across various scientific disciplines has been unknown, as has been the relationship between these biases and reproducibility.
A recent meta-assessment of meta-analyses1 has sought to address this knowledge gap. The research team for the study included John Ioannidis, who is well known for his 2005 paper Why Most Published Research Findings Are False.
Bias patterns and possible influences
The bias patterns that were the focus of the study included:
Small-study effects: Studies that are smaller (of lower precision) might report effect sizes of larger magnitude. This phenomenon could be due to selective reporting of results or to genuine heterogeneity in study design that results in larger effects being detected by smaller studies.
Gray literature bias: Studies might be less likely to be published if they yielded smaller and/or statistically nonsignificant effects and might be therefore only available in PhD theses, conference proceedings, books, personal communications, and other forms of “gray” literature.
Decline effect: The earliest studies to report an effect might overestimate its magnitude relative to later studies, due to a decreasing field-specific publication bias over time or to differences in study design between earlier and later studies.
Early-extreme: An alternative scenario to the decline effect might see earlier studies reporting extreme effects in any direction, because extreme and controversial findings have an early window of opportunity for publication.
Citation bias: The number of citations received by a study might be correlated to the magnitude of effects reported.
US effect: Publications from authors working in the United States might overestimate effect sizes, a difference that could be due to multiple sociological factors.
Industry bias: Industry sponsorship may affect the direction and magnitude of effects reported by biomedical studies. We generalized this hypothesis to nonbiomedical fields by predicting that studies with coauthors affiliated to private companies might be at greater risk of bias.
The sociological and psychological factors commonly seen as underlying these bias patterns include:
Pressures to publish: Scientists subjected to direct or indirect pressures to publish might be more likely to exaggerate the magnitude and importance of their results to secure many high-impact publications and new grants. One type of pressure to publish is induced by national policies that connect publication performance with career advancement and public funding to institutions.
Mutual control: Researchers working in close collaborations are able to mutually control each other’s work and might therefore be less likely to engage in questionable research practices (QRP). If so, risk of bias might be lower in collaborative research but, adjusting for this factor, higher in long-distance collaborations.
Career stage: Early-career researchers might be more likely to engage in QRP, because they are less experienced and have more to gain from taking risks.
Gender of scientist: Males are more likely to take risks to achieve higher status and might therefore be more likely to engage in QRP. This hypothesis was supported by statistics of the US Office of Research Integrity, which, however, may have multiple alternative explanations.
Individual integrity: Narcissism or other psychopathologies underlie misbehavior and unethical decision making and therefore might also affect individual research practices.
Findings
- [The] results consistently suggest that small-study effects, gray literature bias, and citation bias might be the most common and influential issues.
- Small-study effects, in particular, had by far the largest magnitude, suggesting that these are the most important source of bias in meta-analysis, which may be the consequence either of selective reporting of results or of genuine differences in study design between small and large studies.
- [The study] found consistent support for common speculations that, independent of small-study effects, bias is more likely among early-career researchers, those working in small or long-distance collaborations, and those that might be involved with scientific misconduct.
- Choices in study design aimed at maximizing the detection of … [small-study effects] might be justified in some discovery-oriented research contexts.
- [The] results suggest that there is a connection between bias and retractions, offering some support to a responsible conduct of research program that assumes negligence, QRP, and misconduct to be connected phenomena that may be addressed by common interventions.
- [The] results support the notion that mutual control between team members might protect a study from bias.
- [The] findings support the view that a culture of openness and communication, whether fostered at the institutional or the team level, might represent a beneficial influence.
- [The] notion that pressures to publish have a direct effect on bias was not supported and even contrarian evidence was seen: The most prolific researchers and those working in countries where pressures are supposedly higher were significantly less likely to publish overestimated results, suggesting that researchers who publish more may indeed be better scientists and thus report their results more completely and accurately.
- A link between pressures to publish and questionable research practices cannot be excluded, but is likely to be modulated by characteristics of study and authors, including the complexity of methodologies, the career stage of individuals, and the size and distance of collaborations.
- Systematic differences in the risk of bias between physical, biological, and social sciences were observed, particularly for the most prominent biases, as was expected based on previous evidence.
- [The] analysis offered a “bird’s-eye view” of bias in science. It is likely that more complex, fine-grained analyses targeted to specific research fields will be able to detect stronger signals of bias and its causes. However, such results would be hard to generalize and compare across disciplines, which was the main objective of this study.
Source: Ars Technica.
Reference:
- Fanelli, D., Costas, R., & Ioannidis, J. P. (2017). Meta-assessment of bias in science. Proceedings of the National Academy of Sciences, 201618569. ↩
Also published on Medium.