
Transformation from data base to knowledge base: enhancing the value of financial research repositories
In financial market research, repositories are not just stores of information but also wellsprings of insight and wisdom, forming the foundation of efficient knowledge management (KM). As financial technology advances rapidly, high-quality repositories have become essential tools for identifying market trends, discovering investment opportunities, and implementing risk management strategies. The practice of making data-driven decisions by analyzing historical and real-time data to pinpoint undervalued aspects of assets has emerged as a critical competitive edge for financial institutions. Hence, incorporating KM principles into the development and utilization of repositories is of significant importance for fostering financial innovation and enhancing industry efficiency. This approach ensures swift adaptation to market shifts and improves the quality of decision-making.
However, in the practice of the financial industry, the quality of intellectual repository varies significantly, with many still merely in the stage of simple data storage and updating, failing to fully unleash their potential value. This article aims to explore how to transform basic databases into more advanced knowledge bases by applying the DIKW (data-information-knowledge-wisdom) model. Such a transformation requires not only the integration and analysis of data but more importantly, the deep conversion of data into knowledge, thereby providing solid support for financial resolution.
In the DIKW framework, a knowledge base is defined as a system capable of offering in-depth insights and decision support by synthesizing and analyzing data, thus navigating the complex dynamics of the financial market. This article will explore the evolution from simple data repositories to knowledge bases, highlighting the significance of this shift within the current financial sector and outlining the associated challenges and opportunities.
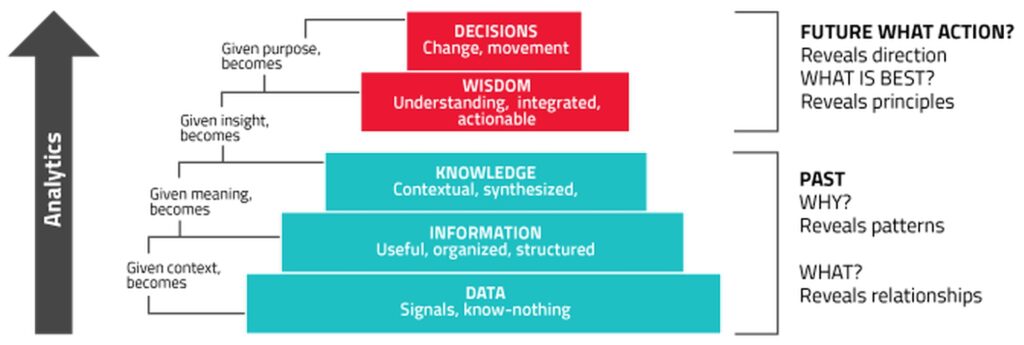
Current analysis of database status
The secondary financial markets are mainly divided into the stock market and the bond market, where the data management needs of the stock and bond markets exhibit some differences. Although both are progressing towards the ideal depicted by the DIKW model (data-information-knowledge-wisdom), reflecting the transformation from data to information level, they struggle to evolve into a knowledge base.
Comparison of data, information, knowledge, wisdom (source: adapted from Scagnetti, 20132).
Data | Information | Knowledge | Wisdom | |
Theoretical understanding | Difficult What is data? |
Easy Information and know-that |
Easy Know-how and tacit often coming from experience |
Easy Context |
Identifying a relevant example | Easy Elements |
Easy Project starting point |
Easy Secret tricks and personal practice |
Difficult Need reflection and meditation |
Visually representing the concept | Difficult Refrain to express context |
Easy Data + visual, saying something about one element |
Easy Instructions and representation of learning |
Easy Fundamentally text based |
Firstly, for the stock market, the databases commonly used by analysts mainly include 1) corporate financial databases and 2) industry databases.
The former’s data comes from quarterly, semi-annual, and annual financial reports of companies, with the primary analysis aimed at forecasting financial performance, conducting valuation, and making investment decisions based on whether the latest financial data misses or beats market expectations.
Despite the availability of APIs for automatic data updates, many financial analysts still prefer manual data entry. The main reason is that third-party data service companies, which import company financial report data through big data processing, occasionally make errors. Financial analysts’ valuation models are personalized, involving business segmentation, peer comparison, etc., and standardized financial report databases often cannot meet their needs. At this point, the repository has evolved from a database to an information base.
The latter, industry databases, often source data from third-party data service companies. Since these are not built by financial analysts themselves, the purpose of data collection is more ambiguous, lacking contextualization, making them more of a database rather than an information base.
Secondly, for the bond market, databases often achieve automatic updates through APIs too, including simple calculations and corrections, as well as some weighted data calculations, thereby condensing the data. As the analysis of key data on bonds has become more standardized, analysts rarely manually input their own. To conclude, this type of database is closer to an information base type.
Transforming into a KMS
To transform into a knowledge management system (KMS), when constructing a financial KMS, it’s essential first to ensure that the database serves not merely as a repository for data but as a structured means to enhance the retrievability and analyzability of data, supporting collaboration and decision-making within teams and organizations. This step aligns with the transition from information to knowledge in the DIKW model, where contextualization, categorization, and computation of data lay the groundwork for uncovering the stories behind the data.
Difference between data base and knowledge base (source: adapted from Tanwar et al., 20103).
Data Base | Knowledge Base | |
1 | Collection of data in database represents the facts. | It consist information at higher level of abstraction. |
2 | Operates on a single object. | Operates on a class of objects. |
3 | Updates are performed by clerical persons. | Updates are performed by domain experts. |
4 | All information needed to be explicitly stated. | Knowledge base has the power of inference. |
Addressing a common issue in financial databases—lack of comparative analysis—can be achieved by integrating historical data with industry benchmarks to enhance the database’s capacity for innovative knowledge. This approach aids in measuring relative performance and discerning potential market trends.
Further, scenario analysis tools like Excel’s Scenario Manager and Solver allow for hypothetical analysis, aiding users in extracting knowledge from information. By constructing financial forecasts under different market conditions, financial data is transformed into practical, advanced knowledge.
Lastly, exploring correlations within the database, such as the relationship between macroeconomic indicators and bond market trends, and connecting different datasets through methods like factor analysis, are crucial steps towards evolving into a mature knowledge base. Such analyses not only enhance the intrinsic value of the data but also provide decision-makers with profound insights in a complex financial environment.
Case of Leveraging knowledge bases to optimize investment management
The background is that an investment bank is faced with the need to identify factors that have an impact on clients and assess the value and risk of these impacts. It was decided to use knowledge graphs and data analysis to improve its risk management capabilities and ensure rapid and accurate identification and assessment of the impact on customers.
Regarding the solution, firstly investment banks build knowledge graphs that cover the relationships between companies and industries, companies and customers, and companies and market prices. This enables banks to analyze problems from a new perspective, namely through corporate group relationships to identify key factors affecting customers.
Regarding big data analysis, investment banks integrate and analyze structured data (such as transaction flow and purchase orders) and unstructured data (including internal bank data, Internet media information and regulatory announcements). Risk events, complete the conversion from raw information to in-depth insights.
Regarding machine learning models, with the help of external third-party institutions, investment banks have developed predictive models, based on insights from big data and knowledge graphs, to achieve the capabilities of risk identification, transmission and prediction. These models can predict market changes that may affect customers by analyzing information such as investment relationships, transaction relationships, and trade relationships.
In terms of results, by combining knowledge graph, big data analysis and machine learning technology, the bank successfully transformed huge data resources into profound business insights and optimized the risk management process. This case shows how financial institutions can use advanced technology to respond to market changes and improve its KM and risk assessment capabilities.
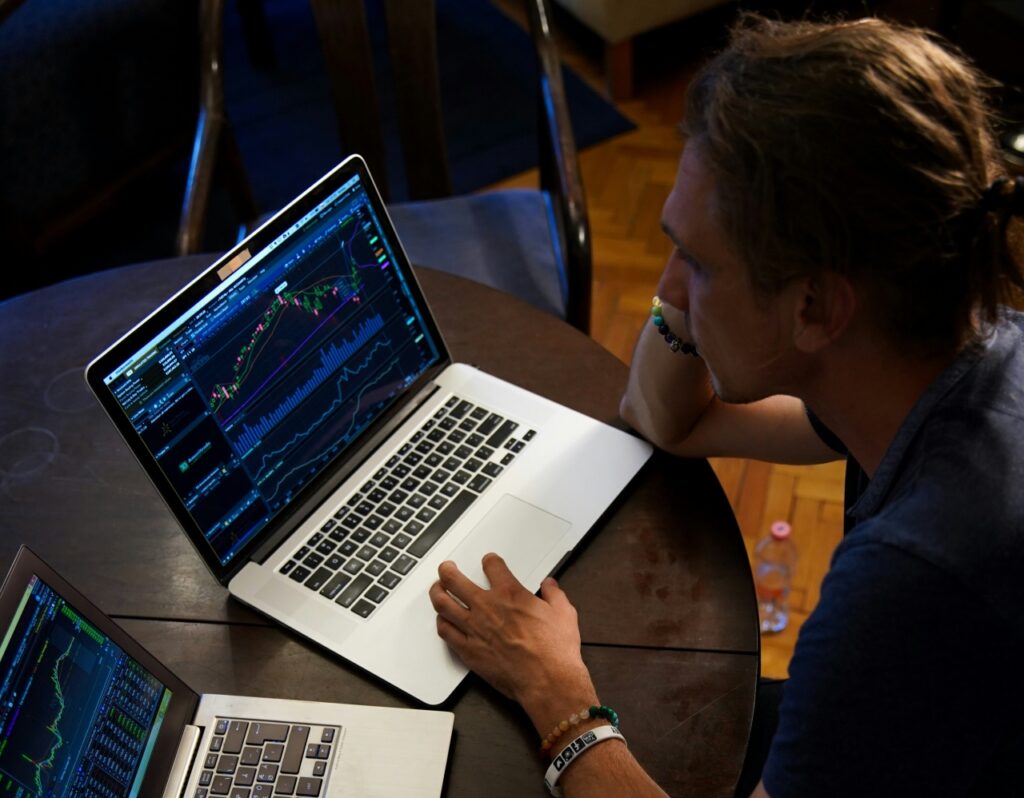
(source: Adam Nowakowski on Unsplash).
Management and cultural aspects
In the field of financial analysis, personalized databases and data drafts constitute an analyst’s core competitiveness, but this personalization brings a certain exclusivity. Although analysts may be reluctant to share these personal knowledge assets, a culture of knowledge sharing can be cultivated through organizational strategies and structural changes. Creating Communities of Practice (CoP) and informal exchange environments can facilitate the flow of knowledge. Moreover, reinforcing the value of knowledge sharing through regular seminars, workshops on sharing best practices, and strategically rewarding those who contribute to the public knowledge base can strengthen the culture of knowledge sharing.
In such an environment, analysts are encouraged to share their database optimization techniques, thereby enhancing the entire organization’s KM capabilities. Through these means, financial institutions can not only enhance their internal knowledge bases but also promote innovation and collaboration on a broader level, ensuring continuous knowledge flow and organizational adaptability.
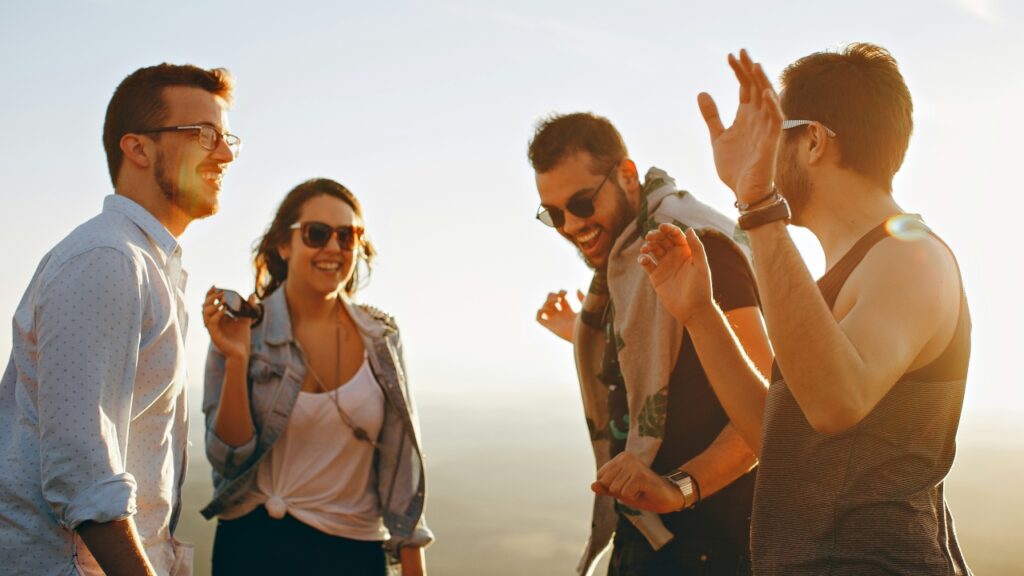
(source: Helena Lopes on Unsplash).
Conclusion
In the finance industry, the construction and application of databases are not only about technical considerations but also an essential part of KM practices. By applying the DIKW model, financial institutions can manage and utilize data more effectively, thereby fostering innovation, transfer, and sharing of knowledge. In summary, integrating KM principles into the construction and application of financial databases is crucial for enhancing the efficiency and innovation capability of the finance industry.
Article source: Adapted from Transformation from Data Base to Knowledge Base: Enhancing the Value of Financial Research Repositories prepared as part of the requirements for completion of course KM6304 Knowledge Management Strategies and Policies in the Nanyang Technological University Singapore Master of Science in Knowledge Management (KM).
Header image source: krakenimages on Unsplash.
References:
- Mannion, P. (2015, January 12). Optimal Analysis Algorithms are IoT’s Big Opportunity. Electronics360, https://electronics360.globalspec.com/article/4890/optimal-analysis-algorithms-are-iot-s-big-opportunity. ↩
- Scagnetti, G. (2013, December 3-4). Searching for Wisdom. Experimenting with Visualization using the DIKW model. DesignEd Asia Conference 2013. https://www.designedasia.com/2013/Full_Papers/B7_SEARCHING%20FOR%20WISDOM.pdf. ↩
- Tanwar, P., Prasad, T. V., & Aswal, M. S. (2010). Comparative study of three declarative knowledge representation techniques. International Journal on Computer Science and Engineering, 2(07), 2274-2281. http://www.enggjournals.com/ijcse/doc/IJCSE10-02-07-53.pdf. ↩